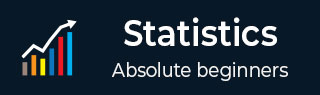
- Statistics Tutorial
- Home
- Adjusted R-Squared
- Analysis of Variance
- Arithmetic Mean
- Arithmetic Median
- Arithmetic Mode
- Arithmetic Range
- Bar Graph
- Best Point Estimation
- Beta Distribution
- Binomial Distribution
- Black-Scholes model
- Boxplots
- Central limit theorem
- Chebyshev's Theorem
- Chi-squared Distribution
- Chi Squared table
- Circular Permutation
- Cluster sampling
- Cohen's kappa coefficient
- Combination
- Combination with replacement
- Comparing plots
- Continuous Uniform Distribution
- Continuous Series Arithmetic Mean
- Continuous Series Arithmetic Median
- Continuous Series Arithmetic Mode
- Cumulative Frequency
- Co-efficient of Variation
- Correlation Co-efficient
- Cumulative plots
- Cumulative Poisson Distribution
- Data collection
- Data collection - Questionaire Designing
- Data collection - Observation
- Data collection - Case Study Method
- Data Patterns
- Deciles Statistics
- Discrete Series Arithmetic Mean
- Discrete Series Arithmetic Median
- Discrete Series Arithmetic Mode
- Dot Plot
- Exponential distribution
- F distribution
- F Test Table
- Factorial
- Frequency Distribution
- Gamma Distribution
- Geometric Mean
- Geometric Probability Distribution
- Goodness of Fit
- Grand Mean
- Gumbel Distribution
- Harmonic Mean
- Harmonic Number
- Harmonic Resonance Frequency
- Histograms
- Hypergeometric Distribution
- Hypothesis testing
- Individual Series Arithmetic Mean
- Individual Series Arithmetic Median
- Individual Series Arithmetic Mode
- Interval Estimation
- Inverse Gamma Distribution
- Kolmogorov Smirnov Test
- Kurtosis
- Laplace Distribution
- Linear regression
- Log Gamma Distribution
- Logistic Regression
- Mcnemar Test
- Mean Deviation
- Means Difference
- Multinomial Distribution
- Negative Binomial Distribution
- Normal Distribution
- Odd and Even Permutation
- One Proportion Z Test
- Outlier Function
- Permutation
- Permutation with Replacement
- Pie Chart
- Poisson Distribution
- Pooled Variance (r)
- Power Calculator
- Probability
- Probability Additive Theorem
- Probability Multiplecative Theorem
- Probability Bayes Theorem
- Probability Density Function
- Process Capability (Cp) & Process Performance (Pp)
- Process Sigma
- Quadratic Regression Equation
- Qualitative Data Vs Quantitative Data
- Quartile Deviation
- Range Rule of Thumb
- Rayleigh Distribution
- Regression Intercept Confidence Interval
- Relative Standard Deviation
- Reliability Coefficient
- Required Sample Size
- Residual analysis
- Residual sum of squares
- Root Mean Square
- Sample planning
- Sampling methods
- Scatterplots
- Shannon Wiener Diversity Index
- Signal to Noise Ratio
- Simple random sampling
- Skewness
- Standard Deviation
- Standard Error ( SE )
- Standard normal table
- Statistical Significance
- Statistics Formulas
- Statistics Notation
- Stem and Leaf Plot
- Stratified sampling
- Student T Test
- Sum of Square
- T-Distribution Table
- Ti 83 Exponential Regression
- Transformations
- Trimmed Mean
- Type I & II Error
- Variance
- Venn Diagram
- Weak Law of Large Numbers
- Z table
- Statistics Useful Resources
- Statistics - Discussion
Statistics - Notations
Following table shows the usage of various symbols used in Statistics
Capitalization
Generally lower case letters represent the sample attributes and capital case letters are used to represent population attributes.
$ P $ - population proportion.
$ p $ - sample proportion.
$ X $ - set of population elements.
$ x $ - set of sample elements.
$ N $ - set of population size.
$ N $ - set of sample size.
Greek Vs Roman letters
Roman letters represent the sample attributs and greek letters are used to represent Population attributes.
$ \mu $ - population mean.
$ \bar x $ - sample mean.
$ \delta $ - standard deviation of a population.
$ s $ - standard deviation of a sample.
Population specific Parameters
Following symbols represent population specific attributes.
$ \mu $ - population mean.
$ \delta $ - standard deviation of a population.
$ {\mu}^2 $ - variance of a population.
$ P $ - proportion of population elements having a particular attribute.
$ Q $ - proportion of population elements having no particular attribute.
$ \rho $ - population correlation coefficient based on all of the elements from a population.
$ N $ - number of elements in a population.
Sample specific Parameters
Following symbols represent population specific attributes.
$ \bar x $ - sample mean.
$ s $ - standard deviation of a sample.
$ {s}^2 $ - variance of a sample.
$ p $ - proportion of sample elements having a particular attribute.
$ q $ - proportion of sample elements having no particular attribute.
$ r $ - population correlation coefficient based on all of the elements from a sample.
$ n $ - number of elements in a sample.
Linear Regression
$ B_0 $ - intercept constant in a population regression line.
$ B_1 $ - regression coefficient in a population regression line.
$ {R}^2 $ - coefficient of determination.
$ b_0 $ - intercept constant in a sample regression line.
$ b_1 $ - regression coefficient in a sample regression line.
$ ^{s}b_1 $ - standard error of the slope of a regression line.
Probability
$ P(A) $ - probability that event A will occur.
$ P(A|B) $ - conditional probability that event A occurs, given that event B has occurred.
$ P(A') $ - probability of the complement of event A.
$ P(A \cap B) $ - probability of the intersection of events A and B.
$ P(A \cup B) $ - probability of the union of events A and B.
$ E(X) $ - expected value of random variable X.
$ b(x; n, P) $ - binomial probability.
$ b*(x; n, P) $ - negative binomial probability.
$ g(x; P) $ - geometric probability.
$ h(x; N, n, k) $ - hypergeometric probability.
Permutation/Combination
$ n! $ - factorial value of n.
$ ^{n}P_r $ - number of permutations of n things taken r at a time.
$ ^{n}C_r $ - number of combinations of n things taken r at a time.
Set
$ A \Cap B $ - intersection of set A and B.
$ A \Cup B $ - union of set A and B.
$ \{ A, B, C \} $ - set of elements consisting of A, B, and C.
$ \emptyset $ - null or empty set.
Hypothesis Testing
$ H_0 $ - null hypothesis.
$ H_1 $ - alternative hypothesis.
$ \alpha $ - significance level.
$ \beta $ - probability of committing a Type II error.
Random Variables
$ Z $ or $ z $ - standardized score, also known as a z score.
$ z_{\alpha} $ - standardized score that has a cumulative probability equal to $ 1 - \alpha $.
$ t_{\alpha} $ - t statistic that has a cumulative probability equal to $ 1 - \alpha $.
$ f_{\alpha} $ - f statistic that has a cumulative probability equal to $ 1 - \alpha $.
$ f_{\alpha}(v_1, v_2) $ - f statistic that has a cumulative probability equal to $ 1 - \alpha $ and $ v_1 $ and $ v_2 $ degrees of freedom.
$ X^2 $ - chi-square statistic.
Summation Symbols
$ \sum $ - summation symbol, used to compute sums over a range of values.
$ \sum x $ or $ \sum x_i $ - sum of a set of n observations. Thus, $ \sum x = x_1 + x_2 + ... + x_n $.