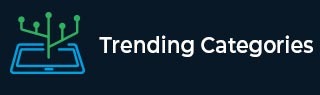
Data Structure
Networking
RDBMS
Operating System
Java
MS Excel
iOS
HTML
CSS
Android
Python
C Programming
C++
C#
MongoDB
MySQL
Javascript
PHP
Physics
Chemistry
Biology
Mathematics
English
Economics
Psychology
Social Studies
Fashion Studies
Legal Studies
- Selected Reading
- UPSC IAS Exams Notes
- Developer's Best Practices
- Questions and Answers
- Effective Resume Writing
- HR Interview Questions
- Computer Glossary
- Who is Who
Properties of linear regression lines
Introduction
In many fields, linear regression is a popular statistical technique for modeling the relationship between two variables. We can use this potent instrument to make predictions based on previous observations. We will talk about the properties of linear regression lines, which are the lines that fit a set of data points the best in this article.
Understanding Properties of linear regression lines
The properties are listed as −
Linearity − Linearity is the first quality of linear regression lines. This indicates that there is a linear relationship between the dependent variable, y, and the independent variable, x. To put it another way, y increases or decreases at the same rate as x does.
Slope − A linear regression line's slope indicates how steep the line is. It tells us how much y changes for every unit change in x. A positive slope indicates that y increases as x increases, while a negative slope indicates that y decreases as x increases. It is defined as the change in y divided by the change in x.
Intercept − When x is zero, the value of y is the intercept of a linear regression line. The point where the line crosses the y-axis is this location. The capture is otherwise called the consistent term.
Residuals − The differences between the predicted and actual y values based on the linear regression line are called residuals. R-Squared: They represent the amount of data variability that cannot be explained by the linear relationship between x and y. A measure of how well the data fits the linear regression line is R-squared. The coefficient of determination is another name. It has values between 0 and 1, with a value of 1 representing a flawless fit.
Normal Error − A measure of how accurate the predictions made by the linear regression line is the standard error of the estimate. It estimates how much variety in the reliant variable that isn't made sense of by the autonomous variable.
Significance − Hypothesis testing can be used to determine whether the linear regression line's slope and intercept are significant. We can conclude that the slope or intercept is statistically significant if the p-value is less than the significance level, which is typically 0.05.
Outliers − Outliers are data points that stand out significantly from the rest of the set. They can altogether affect the direct relapse line, and it is vital to distinguish and eliminate them in the event that they are influencing the precision of the expectations.
Assumptions − Linearity, independence, normality, and homoscedasticity are just a few of the assumptions that linear regression relies on. The linear regression line's predictions may be inaccurate if any of these assumptions are violated.
Multicollinearity − Multicollinearity happens when at least two autonomous factors are exceptionally related with one another. Because of this, it may be challenging to ascertain the distinct effects that each variable has on the dependent variable.
Extrapolation − Predicting values that are outside the range of the independent variable is known as extrapolation. Because it assumes that the linear relationship between x and y continues beyond the data that is observed, it can be risky.
Causality − Straight relapse can show relationship between factors, not causation. It is essential to keep in mind that the fact that two variables have a correlation does not imply that one causes the other.
Choosing a Model − Simple linear regression, multiple linear regression, and polynomial regression are all examples of linear regression models. It is essential to pick the fitting model in light of the idea of the information and the examination question.
Overfitting − Overfitting happens when the direct relapse model is excessively complicated and fits the preparation information too intently, bringing about lackluster showing on new information. Regularization methods like ridge regression and lasso regression can be used to address this.
Underfitting − Underfitting occurs when the linear regression model is too straightforward and fails to account for the intricate nature of the relationship between x and y. As a result, it performs poorly on both new and training data. This can be fixed by using a more complicated model or adding more variables.
Relationships that Aren't Linear The linear relationship between x and y is assumed by linear regression. However, the relationship may not always be linear in some instances. In such cases, nonlinear relapse or other nonlinear demonstrating strategies might be more suitable.
Heteroscedasticity − When the variance of the residuals is not constant over the independent variable's range, heteroscedasticity occurs. This abuses the suspicion of homoscedasticity and can prompt one-sided and wasteful evaluations. Using weighted least squares or transforming the variables can deal with heteroscedasticity.
Autocorrelation − Autocorrelation happens when the residuals are associated with one another. This is against the independence assumption and may result in inaccurate and biased estimates. Time series modeling techniques or including lagged variables in the model can be used to address autocorrelation.
Robustness − Outliers and assumptions that are not met can be problematic for linear regression. To increase the model's robustness, robust regression methods like M-estimation or least trimmed squares can be utilized.
Interpretation − Keeping all other variables constant, the linear regression model's coefficients represent the effect of the independent variable on the dependent variable. It is essential to interpret the coefficients in relation to the research question and to be aware of any potential variables that could cause confusion.
Real-life applications where the properties of linear regressions can be applied
Efficacy of the advertisement − An organization needs to decide the viability of its publicizing effort. They can utilize direct relapse to demonstrate the connection between publicizing use (autonomous variable) and deals income (subordinate variable). The increase in sales revenue for each additional dollar spent on advertising would be represented by the slope of the regression line.
Changes in climate − To comprehend the effects of climate change, scientists want to model the relationship between atmospheric carbon dioxide (an independent variable) and global temperature (a dependent variable). They can estimate the slope of the relationship and make predictions about how temperatures will change in the future based on various levels of carbon dioxide using linear regression.
Stock Costs − An investor wants to use a variety of economic factors, such as interest rates, inflation, and GDP (independent variables), to predict a specific stock's future price (dependent variable). They can model the relationship between these factors and the stock price using multiple linear regression, allowing them to make well-informed investment decisions.
Conclusion
In conclusion, the statistical technique known as linear regression is a common one for modeling the relationship between two variables. Linearity, slope, intercept, residuals, R-squared, significance, outliers, assumptions, multicollinearity, extrapolation, causality, model selection, overfitting, underfitting, nonlinear relationships, heteroscedasticity, autocorrelation, robustness, and interpretation are just a few of the many important properties it possesses. We can use linear regression to make accurate predictions, draw meaningful conclusions from our data, and address potential model issues by comprehending these properties.