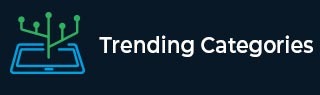
Data Structure
Networking
RDBMS
Operating System
Java
MS Excel
iOS
HTML
CSS
Android
Python
C Programming
C++
C#
MongoDB
MySQL
Javascript
PHP
Physics
Chemistry
Biology
Mathematics
English
Economics
Psychology
Social Studies
Fashion Studies
Legal Studies
- Selected Reading
- UPSC IAS Exams Notes
- Developer's Best Practices
- Questions and Answers
- Effective Resume Writing
- HR Interview Questions
- Computer Glossary
- Who is Who
Advantages and Disadvantages of Linear Regression
Introduction
Linear regression is a broadly utilized factual strategy for modeling and analyzing relationships between variables. It could be a straightforward however capable instrument that permits analysts and examiners to get it the nature of the relationship between a subordinate variable and one or more free factors. However, like several factual method, linear regression has its possess set of points of interest and impediments. In this article, we will investigate these masters and cons to pick up a more profound understanding of when and how to utilize linear regression effectively.
Advantages of Linear Regression
Effortlessness and Interpretability: One of the key focal points of linear regression is its straightforwardness. The strategy is simple to get it and actualize, making it available to both fledglings and experienced examiners. Linear regression produces a straightforward equation that portrays the relationship between the subordinate and free factors. This effortlessness permits analysts to translate the comes about effortlessly and communicate their findings successfully to nontechnical partners.
Linearity Assumption: Linear regression expects a straight relationship between the subordinate and independent factors. Whereas this could appear prohibitive, it can really be beneficial in certain scenarios. When the relationship is around straight, linear regression provides precise and solid comes about. It is especially valuable when the relationship is expected to be straight based on earlier information or theoretical suspicions.
Quantifying Relationships and Making Predictions: Linear regression gives a quantitative degree of the quality and direction of the relationship between factors. The coefficients of the regression condition speak to the alteration within the subordinate variable related to a one−unit alteration within the autonomous variable(s). This empowers analysts to evaluate the effect of distinctive factors on the result of intrigued and make forecasts based on the demonstration.
Model Interpretation and Variable Determination: Linear regression allows analysts to distinguish the foremost persuasive factors within the demonstration. By analyzing the size and noteworthiness of the coefficients, analysts can decide which free factors have the most grounded effect on the dependent variable. This data helps in variable choice, directing the incorporation or prohibition of indicators within the show.
Diagnostic Tools: Linear regression gives an extent of demonstrative apparatuses to evaluate the quality of the show and recognize potential issues. Leftover examination, for illustration, makes a difference distinguish any deviations from the suspicions of the linear regression model, such as non−linearity, heteroscedasticity, or persuasive exceptions. These demonstrative devices offer assistance to analysts evaluate the validity and unwavering quality of their discoveries.
Drawbacks of Linear Regression
Linear Assumption: The linearity presumption of linear regression can be a disadvantage when the genuine relationship between factors is non-linear. In case the relationship takes after a bent or complex pattern, linear regression may not capture it accurately. In such cases, elective models, such as polynomial regression or non−linear regression, may be more suitable to capture the basic structure of the information.
Sensitivity to Exceptions: Linear regression is delicate to exceptions, which are extraordinary observations that go astray essentially from the common design of the information. Exceptions can excessively impact the regression coefficients and affect the general fit of the demonstration. In this manner, it is pivotal to recognize and address exceptions suitably to avoid them from mutilating the comes about.
Independence Presumption: Linear regression accepts that the perceptions are free of each other. This suspicion suggests that there's no correlation or reliance between the mistakes or residuals of the demonstration. Damaging this presumption can lead to biased and inefficient estimates. It is fundamental to confirm the freedom suspicion by examining the autocorrelation of residuals or utilizing time series strategies in the event that the information displays temporal dependence.
Limited to Linear Relationships: As the title recommends, linear regression models are constrained to capturing straight connections between factors. They cannot viably show non−linear or complex relationships without additional transformations or consolidating non−linear terms into the show. In cases where the relationship is intrinsically non−linear, utilizing linear regression may result in wrong forecasts and deceiving translations.
Multicollinearity: Multicollinearity can too make it challenging to decide the personal commitments of related indicators. Cautious variable determination or procedures like ridge regression or foremost component analysis can moderate multicollinearity−related issues.
Conclusion
Linear regression may be a flexible and important tool in measurable analysis, offering a few advantages for understanding relationships between factors, making forecasts, and translating comes about. Its straightforwardness and interpretability make it a well−known choice, particularly when linearity presumptions hold. In any case, it is vital to consider its restrictions and potential disadvantages. Non−linearity, sensitivity to exceptions, freedom presumptions, constrained modeling capabilities for complex connections, and multicollinearity are variables that ought to be carefully assessed when applying linear regression. By understanding these pros and cons, analysts can make educated choices around when to utilize linear regression and when alternative methods might be more appropriate for their particular research questions and data.