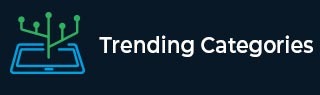
Data Structure
Networking
RDBMS
Operating System
Java
MS Excel
iOS
HTML
CSS
Android
Python
C Programming
C++
C#
MongoDB
MySQL
Javascript
PHP
Physics
Chemistry
Biology
Mathematics
English
Economics
Psychology
Social Studies
Fashion Studies
Legal Studies
- Selected Reading
- UPSC IAS Exams Notes
- Developer's Best Practices
- Questions and Answers
- Effective Resume Writing
- HR Interview Questions
- Computer Glossary
- Who is Who
What is Regression?
Regression defines a type of supervised machine learning approaches that can be used to forecast any continuous-valued attribute. Regression provides some business organization to explore the target variable and predictor variable associations. It is an essential tool to explore the data that can be used for monetary forecasting and time series modeling.
Data can be smoothed by fitting the data to a function, such as with regression. Linear regression includes discovering the “best” line to fit two attributes (or variables) therefore that one attribute can be used to predict the other. Several linear regression is an advancement of linear regression, where higher than two attributes are included and the data are fit to a multidimensional space.
In linear regression, the data are modeled to fit a straight line. For example, a random variable, y (called response variable), can be modeled as a linear function of another random variable, x (called a predictor variable), with the equation y = wx+b, where the variance of y is considered to be constant.
Regression issues manage with the computation of an output value placed on input values. When used for classification, the input values are values from the database and the output values represent the classes. Regression can be used to explore classification problems, but it can be used for multiple applications such as forecasting. The elementary structure of regression is simple linear regression that contains only one predictor and a prediction.
Regression can be used to perform classification using two methods which are as follows −
Division − The data are divided into regions situated on class.
Prediction − Formulas are produced to predict the output class’s value.
These methods are used to predict the value of a response (dependent) variable from one or more predictor (independent) variables where the variables are integer. There are multiple forms of regression, such as linear, multiple, weighted, polynomial, nonparametric, and robust (robust techniques are useful when errors fail to need normalcy conditions or when the data contains significant outliers).
Regression can predict some dependent data sets, defined in the expression of separate variables, and the trend is accessible for a definite period. Regression supports a good method to predict variables, but there are specific restrictions and assumptions such as the independence of the variables, inherent normal distributions of the variables.
Each regression tree leaf stores a continuous-valued prediction, which is the average cost of the predicted attribute for the training sets that cover the leaf. By contrast, in model trees, each leaf tends to a regression model and a multivariate continuous equation for the predicted attribute. Regression and model trees influence to be effective than linear regression when the data are not represented well by an easy linear model.
To Continue Learning Please Login