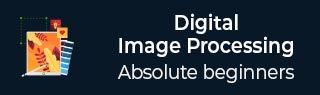
- Digital Image Processing
- DIP - Home
- DIP - Image Processing Introduction
- DIP - Signal and System Introduction
- DIP - History of Photography
- DIP - Applications and Usage
- DIP - Concept of Dimensions
- DIP - Image Formation on Camera
- DIP - Camera Mechanism
- DIP - Concept of Pixel
- DIP - Perspective Transformation
- DIP - Concept of Bits Per Pixel
- DIP - Types of Images
- DIP - Color Codes Conversion
- DIP - Grayscale to RGB Conversion
- DIP - Concept of Sampling
- DIP - Pixel Resolution
- DIP - Concept of Zooming
- DIP - Zooming methods
- DIP - Spatial Resolution
- DIP - Pixels Dots and Lines per inch
- DIP - Gray Level Resolution
- DIP - Concept of Quantization
- DIP - ISO Preference curves
- DIP - Concept of Dithering
- DIP - Histograms Introduction
- DIP - Brightness and Contrast
- DIP - Image Transformations
- DIP - Histogram Sliding
- DIP - Histogram Stretching
- DIP - Introduction to Probability
- DIP - Histogram Equalization
- DIP - Gray Level Transformations
- DIP - Concept of convolution
- DIP - Concept of Masks
- DIP - Concept of Blurring
- DIP - Concept of Edge Detection
- DIP - Prewitt Operator
- DIP - Sobel operator
- DIP - Robinson Compass Mask
- DIP - Krisch Compass Mask
- DIP - Laplacian Operator
- DIP - Frequency Domain Analysis
- DIP - Fourier series and Transform
- DIP - Convolution theorm
- DIP - High Pass vs Low Pass Filters
- DIP - Introduction to Color Spaces
- DIP - JPEG compression
- DIP - Optical Character Recognition
- DIP - Computer Vision and Graphics
- DIP Useful Resources
- DIP - Quick Guide
- DIP - Useful Resources
- DIP - Discussion
Introduction to Frequency domain
We have deal with images in many domains. Now we are processing signals (images) in frequency domain. Since this Fourier series and frequency domain is purely mathematics, so we will try to minimize that math’s part and focus more on its use in DIP.
Frequency domain analysis
Till now, all the domains in which we have analyzed a signal , we analyze it with respect to time. But in frequency domain we don’t analyze signal with respect to time, but with respect of frequency.
Difference between spatial domain and frequency domain
In spatial domain, we deal with images as it is. The value of the pixels of the image change with respect to scene. Whereas in frequency domain, we deal with the rate at which the pixel values are changing in spatial domain.
For simplicity, Let’s put it this way.
Spatial domain
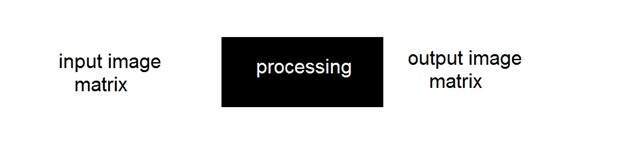
In simple spatial domain, we directly deal with the image matrix. Whereas in frequency domain, we deal an image like this.
Frequency Domain
We first transform the image to its frequency distribution. Then our black box system perform what ever processing it has to performed, and the output of the black box in this case is not an image, but a transformation. After performing inverse transformation, it is converted into an image which is then viewed in spatial domain.
It can be pictorially viewed as
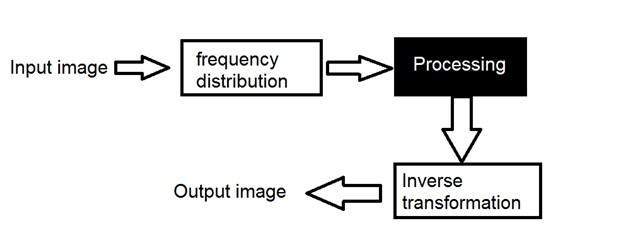
Here we have used the word transformation. What does it actually mean?
Transformation
A signal can be converted from time domain into frequency domain using mathematical operators called transforms. There are many kind of transformation that does this. Some of them are given below.
- Fourier Series
- Fourier transformation
- Laplace transform
- Z transform
Out of all these, we will thoroughly discuss Fourier series and Fourier transformation in our next tutorial.
Frequency components
Any image in spatial domain can be represented in a frequency domain. But what do this frequencies actually mean.
We will divide frequency components into two major components.
High frequency components
High frequency components correspond to edges in an image.
Low frequency components
Low frequency components in an image correspond to smooth regions.
To Continue Learning Please Login