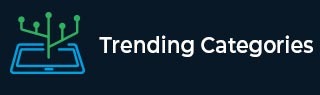
Data Structure
Networking
RDBMS
Operating System
Java
MS Excel
iOS
HTML
CSS
Android
Python
C Programming
C++
C#
MongoDB
MySQL
Javascript
PHP
Physics
Chemistry
Biology
Mathematics
English
Economics
Psychology
Social Studies
Fashion Studies
Legal Studies
- Selected Reading
- UPSC IAS Exams Notes
- Developer's Best Practices
- Questions and Answers
- Effective Resume Writing
- HR Interview Questions
- Computer Glossary
- Who is Who
How Does Removing the Intercept Term for Improvement Work?
Introduction
Regression analysis is a common statistical method for simulating the relationship between a dependent variable and one or more independent variables. When all the independent variables are equal to zero, the expected value of the dependent variable is represented by the intercept term in a regression equation. But dropping the intercept term occasionally might result in more precise regression results and higher model performance. The idea of intercept removal in regression analysis will be discussed in this article, along with its advantages, disadvantages, and implications for interpreting regression data.
What is Intercept Removal?
Regression analysis employs the statistical method of intercept removal, often referred to as intercept centering, to modify the intercept term of a regression equation. The intercept term in a straightforward linear regression equation denotes the value of the dependent variable that is anticipated when the independent variable equals zero. Intercept removal effectively centers the data around zero by subtracting the dependent variable's mean from each observation.
Instead of having an intercept term estimated from the data, the intercept removal has the effect of forcing the regression line to pass through the origin (0,0) on the scatterplot of the data. This can be helpful in circumstances where it's crucial to eliminate a constant term's impact on the regression equation.
Intercept removal can be done in several different ways depending on the analysis tool utilized. Intercept removal can be accomplished by inserting the argument "0" or "-1" in the calculation for the regression model in several statistical tools, such as R or Python. The software is instructed to remove the intercept term from the regression equation via this. The dependent variable's mean can also be subtracted from each observation before the regression analysis is done to remove the intercept.
It's important to remember that intercept removal is only sometimes suitable or necessary in a regression analysis. It is often beneficial to keep the intercept term because it tells us a lot about the baseline value of the dependent variable. Additionally, removing the intercept from the regression equation occasionally results in biased estimates and increased collinearity between the independent variables. The specific qualities of the data and the research issue under investigation should be considered when deciding whether to keep or eliminate the intercept term in a regression analysis.
Benefits of Intercept Removal
There are various possible advantages of intercept removal for regression analysis. Firstly, eliminating the intercept term can lessen the influence of outliers on the regression estimates. Outliers are observations that stand out from the rest of the data significantly. They can skew the results of a regression, especially if the intercept term is present. The regression coefficients are less susceptible to outliers when the data is centered around zero because the value of the intercept term is no longer a factor.
Secondly, deleting the intercept term can make the regression coefficients easier to understand. The coefficients, which reflect the change in the dependent variable for a unit change in the independent variable, holding all other variables constant, might need help understanding when the intercept term is present in the regression equation. The coefficients, on the other hand, show the change in the dependent variable for a unit change in the independent variable relative to the mean of the dependent variable when the intercept factor is eliminated. In cases where the mean of the dependent variable has a valid interpretation, this interpretation may be more logical.
Thirdly, when the dependent variable is centered around zero, intercept removal can help increase the regression estimations' precision. When the intercept term is included in the regression equation, it is assumed that even when all independent variables are equal to 0, the value of the dependent variable is not zero. The intercept term, however, might induce bias in the regression estimations when the dependent variable is naturally centered around zero, as in the case of standardized variables. The regression estimations are more precise when the intercept term is eliminated because its value no longer impacts them.
Drawbacks of Intercept Removal
Despite the potential benefits of intercept removal, several disadvantages need to be taken into consideration −
The expected value of the dependent variable when all the independent variables are equal to zero is represented by the intercept term, a component of the regression equation. This is a useful starting point for analyzing the regression coefficients. Interpretation of coefficients might be challenging because of the removal of the intercept term, which is one of the main disadvantages of this method. The regression coefficients, however, show the change in the dependent variable relative to some arbitrary point when the intercept factor is eliminated. For non-experts, this can make it challenging to understand the coefficients meaningfully.
Collinearity between the independent variables in the regression equation might also rise if the intercept term is removed. Collinearity is a statistical phenomenon with a strong correlation between two or more independent variables. This makes it challenging to quantify the individual effects of each independent variable on the dependent variable. By establishing a baseline level of the dependent variable unaffected by the independent variables, the intercept term, when present in the regression equation, can aid in reducing collinearity. On the other hand, the independent variables can become more colinear when the intercept factor is removed, which could cause instability in the regression estimations.
Biased estimates − Removing the intercept term can also produce inaccurate regression estimates when the dependent variable is not centered around zero. When the intercept term is eliminated, the regression coefficients show how the dependent variable changes to its mean. Removing the intercept component, however, can inject bias into the regression estimates when the dependent variable has a meaningful interpretation at zero, such as income or age. It could be preferable in certain circumstances to keep the intercept term in the regression equation. This provides a meaningful baseline for interpreting the regression coefficients.
Information loss − Some data-related information could be lost when the intercept term is eliminated. The baseline level of the dependent variable and the total size of the influence of the independent variables, for instance, can both be revealed by the intercept term. It could be more challenging to compare the outcomes of several regression models if the intercept term is removed, especially if the intercept terms differ.
While removing the intercept factor provides a number of advantages that should be carefully evaluated, including lessening the impact of outliers and increasing the precision of the regression estimates, it also has a number of disadvantages. Whether to eliminate or keep the intercept term in a regression analysis should be based on the details of the data and the research issue under consideration. It should be made after carefully weighing this approach's potential advantages and disadvantages.
Implications for Regression Analysis
The interpretation of the regression results and the precision of the regression estimates can be impacted by intercept removal, which has various consequences for regression analysis.
Outliers − When the existence of outliers is skewing the regression findings, intercept removal may be very helpful. It can be challenging to interpret the regression coefficients in these cases since the outliers may significantly impact the intercept term. By removing the impact of outliers on the regression results, intercept reduction can increase the precision of the regression estimations.
Model comparison − Comparing the outcomes of several regression models can be challenging when the intercept is removed. Deciding whether a model offers a better fit for the data can be challenging when the intercept component is present in one model but absent from another. It may be challenging to compare in the findings since the two models may have different interpretations of the regression coefficients.
Conclusion
In conclusion, intercept removal can be a powerful method in regression analysis that offers more modeling flexibility and precision for the relationship between the dependent and independent variables. Although this method can introduce bias, boost collinearity among the independent variables, and make it more challenging to understand the regression results, it must be used with caution. In a regression analysis, whether to keep or remove the intercept term should be based on a comprehensive review of the unique properties of the data and the research issue under investigation. Researcher decisions regarding the regression model to employ and how to interpret the findings can be more effectively made by clearly understanding the advantages and disadvantages of intercept removal.