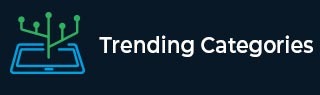
Data Structure
Networking
RDBMS
Operating System
Java
MS Excel
iOS
HTML
CSS
Android
Python
C Programming
C++
C#
MongoDB
MySQL
Javascript
PHP
Physics
Chemistry
Biology
Mathematics
English
Economics
Psychology
Social Studies
Fashion Studies
Legal Studies
- Selected Reading
- UPSC IAS Exams Notes
- Developer's Best Practices
- Questions and Answers
- Effective Resume Writing
- HR Interview Questions
- Computer Glossary
- Who is Who
How can Tensorflow text be used with whitespace tokenizer in Python?
Tensorflow text can be used with whitespace tokenizer by calling the ‘WhitespaceTokenizer’’, which creates a tokenizer, that is used with the ‘tokenize’ method on the string.
Read More: What is TensorFlow and how Keras work with TensorFlow to create Neural Networks?
We will use the Keras Sequential API, which is helpful in building a sequential model that is used to work with a plain stack of layers, where every layer has exactly one input tensor and one output tensor.
A neural network that contains at least one layer is known as a convolutional layer. We can use the Convolutional Neural Network to build learning model.
TensorFlow Text contains collection of text related classes and ops that can be used with TensorFlow 2.0. The TensorFlow Text can be used to preprocess sequence modelling.
We are using the Google Colaboratory to run the below code. Google Colab or Colaboratory helps run Python code over the browser and requires zero configuration and free access to GPUs (Graphical Processing Units). Colaboratory has been built on top of Jupyter Notebook.
Tokenization is the method of breaking down a string into tokens. These tokens can be words, numbers, or punctuation. The key interfaces include Tokenizer and TokenizerWithOffsets each of which have a single method tokenize and tokenize_with_offsets respectively. There are multiple tokenizers, each of which implement TokenizerWithOffsets (which extends the Tokenizer class). This includes an option to get byte offsets into the original string. This helps know the bytes in the original string the token was created from.
N-grams are sequential words when a sliding window of size n is given. When tokens are combined, three reduction mechanisms are supported. For text, Reduction.STRING_JOIN can be used. It appends the strings to each other. The default separator character is space, but can be changed with the string_separater argument.
The other reduction methods are used with numerical values, and they are Reduction.SUM and Reduction.MEAN.
Example
print("Whitespace tokenizer is being called") tokenizer = text.WhitespaceTokenizer() tokens = tokenizer.tokenize(['Everything not saved will be lost.', u'Sad☹'.encode('UTF-8')]) print("Here, n is 2, hence it is bigram") bigrams = text.ngrams(tokens, 2, reduction_type=text.Reduction.STRING_JOIN) print("The bigrams are converted to a list") print(bigrams.to_list())
Output
Whitespace tokenizer is being called Here, n is 2, hence it is bigram The bigrams are converted to a list [[b'Everything not', b'not saved', b'saved will', b'will be', b'be lost.'], []]
Explanation
- The whitespace tokenizer is called.
- The value of ‘n’ is set to 2, hence it is known as a bigram.
- The tokens are stored in a list, and displayed on a console.