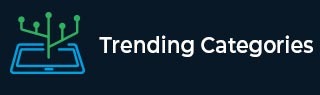
Data Structure
Networking
RDBMS
Operating System
Java
MS Excel
iOS
HTML
CSS
Android
Python
C Programming
C++
C#
MongoDB
MySQL
Javascript
PHP
Physics
Chemistry
Biology
Mathematics
English
Economics
Psychology
Social Studies
Fashion Studies
Legal Studies
- Selected Reading
- UPSC IAS Exams Notes
- Developer's Best Practices
- Questions and Answers
- Effective Resume Writing
- HR Interview Questions
- Computer Glossary
- Who is Who
What are Sampling-Based Approaches?
Sampling is a broadly used method for handling the class imbalance problem. The concept of sampling is to change the distribution of examples so that the rare class is well defined in the training set. There are various techniques for sampling such as undersampling, oversampling, and a hybrid of both approaches. For example, consider a data set that includes 100 positive examples and 1000 negative examples.
In the method of undersampling, a random sample of 100 negative examples is selected to form the training set ahead with all the positive examples. One issue with this method is that some of the helpful negative examples cannot be selected for training, hence, resulting in a less than the optimal model.
The method is to overcome this problem is to implement undersampling multiple times and to induce multiple classifiers same to the ensemble Iearning approach. Focused undersampling methods can be used, where the sampling process creates an informed choice concerning the negative examples that should be removed, e.g., those situated far over from the decision boundary.
Oversampling reflects the positive examples until the training set has the same number of positive and negative examples. The effect of oversampling on the development of a decision boundary using a classifier including a decision tree. The positive example is misclassified because there are not adequate examples to validate the formation of a new decision boundary to independent the positive and negative instances.
But for noisy information, oversampling can generate model overfitting because several noise examples can be replicated multiple times. Oversampling does not insert some new data into the training set. Replication of positive examples avoids the learning algorithm from pruning specific parts of the model that defines regions that include some training examples (i.e., the small disjuncts). The more positive examples also influence the enhancement of the computation time for model building.
The hybrid method needs a set of undersampling the majority class and oversampling the rare class to implement uniform class distribution. Undersampling can be implemented using random or focused subsampling. Oversampling can be done by replicating the current positive examples or producing new positive examples in the neighborhood of the current positive examples.
To Continue Learning Please Login