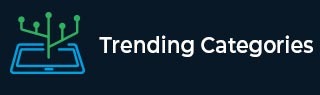
Data Structure
Networking
RDBMS
Operating System
Java
MS Excel
iOS
HTML
CSS
Android
Python
C Programming
C++
C#
MongoDB
MySQL
Javascript
PHP
Physics
Chemistry
Biology
Mathematics
English
Economics
Psychology
Social Studies
Fashion Studies
Legal Studies
- Selected Reading
- UPSC IAS Exams Notes
- Developer's Best Practices
- Questions and Answers
- Effective Resume Writing
- HR Interview Questions
- Computer Glossary
- Who is Who
Sampling Error
Introduction
In any business or research activity, a large number of data is produced, which becomes difficult to analyse. In this direction, a statistical process, sampling, is useful to analyse the entire population. However, in doing so, some error arises due to various reasons. For accurate data analysis, one must know about sampling and the various types of error associated with it. In this tutorial, we will discuss sampling, sampling errors, a basic formula, properties, and ways to minimize sampling error with solved examples.
Sampling
In statistics, sampling is defined as the process of selection of specific data from a large population. It represents the whole population. To make a statistical interference about a large population, it is quite difficult to select data of each entity belonging to the group. In this scenario, the sampling methods help to select an accurate sample for an effective analysis. The sampling methods are widely classified into two categories, namely probability and non-probability sampling methods. The detailed classification of sampling methods is summarized in the following table.
Probability sampling | Non-probability sampling |
---|---|
Simple random | Convenience |
Cluster | Judgemental |
Systematic | Snowball |
Stratified random | Quota |
Various factors affect the sampling process, which is summarized below.
Characteristics and property of the frame
Availability of auxiliary information
Accuracy
Operating cost
Sampling Error
In statistics, sampling error is the difference between the sample statistic and the population parameter it predicts. It is very important to consider during the statistical analysis of a sample to represent the whole population.For example, we want to know the average weight of teenagers in India. Therefore, we collect the weight of teenagers in a state and found that the average weight is 50 kg. In, this case sample mean represents the population means. However, it is not necessary that the sample mean will be equal to the population mean. The deviation between the means is known as sampling error. There are usually four errors occurred in a survey.
Population specification error − This type of error arises when the subject of the survey is unknown. For example, we have to survey kids’ clothes. However, the choice of kids' clothes depends on either of their parents.
Sample frame error − This type of error arises when a wrong sample is collected from a whole population.
Selection error − This error occurs when respondents self-select themselves to participate in this study.
Sampling error − This type of error occurs when there is a discrepancy between the respondents.
Formula
The sampling error formula represents the statistical error, which arises due to the difference between the sample statistic and the population parameter it predicts. Mathematically, it can be expressed as
$$\mathrm{Sampling\:error\:=\:Z\:\times\:\frac{\sigma}{\sqrt{m}}}$$
where Z, 𝜎, and m represent the score value based on confidence level, standard deviation of population, and sample size.
For statistical accuracy, the sampling must be carefully done to avoid unnecessary errors. The following points should be followed to find the sampling error.
Collect all the population data; calculate the population's means and variance.
We should determine the sample size in such a way that it should not be greater than the population size.
In the next step, we need to evaluate the confidence level and determine the Z-score value.
Now, using the sampling formula, we can easily get the value of the sampling error.
Properties
There are various properties of sampling error, which are summarized below.
The sampling error should be unbiased.
The sampling error should be small.
The sample statistics estimate the relationship and effects on the population.
The average or expected value of multiple attempts should equal the population value.
Sources Sampling Error
The sampling error or bias occurs if the sampling statistics don't match the population estimate. There are several reasons for the sampling biases that are described below.
If the sample doesn't represent the whole population
If a wrong sample is collected
If the subjects with specific characteristics don't respond. It is known as a non-response error.
If the measurements don't reflect the population estimates. It is known as measurement error.
How to Reduce Sampling Error?
There are various ways to reduce the sampling error. Some of them are illustrated below.
Increase the sample size: The statistical parameters of a larger sample size are close to the population estimates.
It is recommended to divide the population into groups to reduce sampling biases.
It is necessary to know the population.
We can perform an external record check.
We should be careful while designing the sample.
WE should randomly select the sample.
Solved Examples
Example 1
Let’s consider a survey that over 4000 people have conducted. The standard deviation of the population is 0.25. The confidence level of the population is 95 %. Evaluate the sampling error.
Solution −
According to the question
The sample size $\mathrm{=\:m\:=\:4000}$
The standard deviation is $\mathrm{=\:\sigma\:=\:0.25}$
The value of Z at confidence level 95 % is $\mathrm{=\:Z\:=1.96}$
Using the sampling error formula
$\mathrm{Sampling\:error\:=\:Z\:\times\:\frac{\sigma}{\sqrt{m}}\:=\:1.96\:\times\:\frac{0.25}{\sqrt{4000}}}$
Sampling error $\mathrm{=\:0.0007}$
∴ The sampling error is 0.007.
Example 2
Evaluate the sampling error if the sample size is 300 and the standard deviation of the population is 0.56. The confidence level of the population is 90 %.
Solution −
According to the question,
The sample size $\mathrm{=\:m\:=\:300}$
The standard deviation is $\mathrm{=\:\sigma\:=\:0.56}$
The value of Z at confidence level 90 % is $\mathrm{=\:Z\:=\:1.645}$
Using the sampling error formula,
$\mathrm{Sampling\:error\:=\:Z\:\times\:\frac{\sigma}{\sqrt{m}}\:=\:1.645\:\times\:\frac{0.56}{\sqrt{300}}}$
$\mathrm{Sampling\:error\:=\:0.052}$
∴ The sampling error is 0.053.
Conclusion
The present tutorial gives a brief introduction about sampling errors. The basic meaning of sampling, sampling error, and their properties have been briefly described. In addition, the procedure to minimize the sampling error has been mentioned in this tutorial. Moreover, some solved examples have been provided for better clarity of this concept. In conclusion, the present tutorial may be useful for understanding sampling errors.
FAQs
1. What is the significance of the sample size in sampling error?
The sample size plays an important role in sampling error. It is inversely proportional to sampling error. Therefore, it is always recommended to use a large sample size.
2. What do you mean by confidence interval?
The confidence interval is defined as a range of numerical values that contains the population parameter. In statistics, it is another representation way of probability.
3. What are the types of sampling techniques?
The sampling techniques are widely classified into two categories, namely probability and non-probability sampling methods.
4. What are the types of sampling errors?
There are several types of sampling errors studied in statistics
Population-specific
Selection
Sample frame
Non-response
5. Is sampling error unavoidable?
Yes, the sampling error is unavoidable. The sampling error is the difference between the sample statistics with the population parameter. There is always a small margin of error exist between these two.