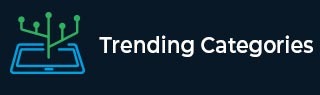
Data Structure
Networking
RDBMS
Operating System
Java
MS Excel
iOS
HTML
CSS
Android
Python
C Programming
C++
C#
MongoDB
MySQL
Javascript
PHP
Physics
Chemistry
Biology
Mathematics
English
Economics
Psychology
Social Studies
Fashion Studies
Legal Studies
- Selected Reading
- UPSC IAS Exams Notes
- Developer's Best Practices
- Questions and Answers
- Effective Resume Writing
- HR Interview Questions
- Computer Glossary
- Who is Who
What is Edge Analytics in IoT?
Edge analytics is a cutting−edge form of data analysis that takes place at the network's edge, where data is actually produced. Unlike traditional analytics conducted in centralized data centers, edge analytics recognizes the growing significance of the Internet of Things (IoT). With the proliferation of IoT devices generating massive data volumes, it becomes impractical or unfeasible to transmit all this information to a central location for analysis. Edge analytics offers a solution by performing data analysis directly at the edge of the network, enabling enhanced performance, reduced latency, and improved security.
In this article, we will discuss more about edge analytics in IoT. Let’s start.
Edge Analytics with IoT Benefits
Edge analytics has a number of benefits for IoT applications. These benefits include:
Reduced Latency: By analyzing data at the edge of the network, near the source of data generation, edge analytics minimizes the time required to process and respond to critical events. This is particularly important in real−time applications where immediate actions or decisions are necessary, such as industrial automation, autonomous vehicles, or remote monitoring of vital systems.
Improved Performance: Edge analytics allows for localized data processing and decision−making. By analyzing and filtering data at the edge, only relevant information is transmitted to the central system, reducing bandwidth usage and improving overall system performance. This optimization can enhance the scalability and efficiency of IoT deployments.
Enhanced Security: Transmitting large volumes of raw data from IoT devices to a centralized location can raise security concerns. Edge analytics mitigates this risk by performing data analysis and filtering at the edge. Sensitive information can be processed and anonymized locally, reducing the exposure of critical data during transmission. This approach helps protect privacy, comply with regulations, and strengthen overall security posture.
Bandwidth Optimization: IoT devices often generate enormous amounts of data, which can strain network resources if sent to a centralized location for analysis. Edge analytics alleviates this strain by performing preliminary analysis and filtering at the edge. Only summarized or relevant data is then transmitted to the central system, optimizing bandwidth utilization and reducing costs.
Offline Operation: In scenarios where the IoT devices operate in remote or disconnected environments with limited or intermittent connectivity, edge analytics enables local processing and decision−making. This capability allows critical operations to continue even when network connectivity is temporarily unavailable, ensuring uninterrupted functionality and improving system resilience.
Real−time Insights: Edge analytics enables rapid analysis and decision−making at the source of data generation. This facilitates immediate response to events, enabling real−time insights and actions. This is particularly beneficial in timesensitive applications such as predictive maintenance, anomaly detection, and emergency response systems.
Overall, edge analytics empowers IoT deployments by providing faster response times, improved performance, enhanced security, optimized bandwidth usage, offline operation capabilities, and real−time insights, all of which contribute to more efficient and effective utilization of IoT data.
Few Cases of Edge Analytics in IoT
Edge analytics in IoT can be applied to various use cases across different industries. Here are some examples:
Predictive Maintenance: By analyzing sensor data at the edge, predictive maintenance algorithms can detect patterns and anomalies in real−time. This allows for proactive maintenance of equipment and machinery, minimizing downtime and optimizing maintenance schedules. Edge analytics enables local processing and immediate action, reducing the need for data transmission to a central system.
Smart Manufacturing: Edge analytics in manufacturing environments enables real−time monitoring and optimization of production processes. By analyzing sensor data from machinery and equipment at the edge, manufacturers can identify inefficiencies, detect quality issues, and optimize workflows. This leads to increased productivity, reduced waste, and improved overall operational efficiency.
Autonomous Vehicles: Edge analytics plays a critical role in enabling real−time decision−making in autonomous vehicles. By analyzing data from multiple sensors, including cameras, LiDAR, and radar, at the edge, vehicles can detect objects, identify hazards, and make split−second decisions without relying heavily on cloud connectivity. This enhances safety, responsiveness, and reduces reliance on constant network connectivity.
Smart Cities: Edge analytics is instrumental in powering various smart city applications. For instance, intelligent traffic management systems can analyze data from traffic sensors, cameras, and other sources at the edge to optimize traffic flow, detect accidents, and manage congestion in real−time. Similarly, smart lighting systems can adjust lighting levels based on local conditions, reducing energy consumption and enhancing safety.
Healthcare Monitoring: Edge analytics enables real−time analysis of patient health data collected from wearable devices and medical sensors. This allows for immediate detection of abnormalities, timely alerts, and faster response to critical situations. Edge analytics also supports remote patient monitoring, where data is processed locally and transmitted to healthcare providers only when necessary, ensuring patient privacy and reducing network bandwidth requirements.
Conclusion
These use cases demonstrate the versatility of edge analytics in IoT, providing localized processing, real−time insights, reduced latency, and improved operational efficiency across a range of industries and applications.