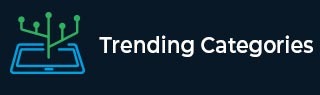
Data Structure
Networking
RDBMS
Operating System
Java
MS Excel
iOS
HTML
CSS
Android
Python
C Programming
C++
C#
MongoDB
MySQL
Javascript
PHP
Physics
Chemistry
Biology
Mathematics
English
Economics
Psychology
Social Studies
Fashion Studies
Legal Studies
- Selected Reading
- UPSC IAS Exams Notes
- Developer's Best Practices
- Questions and Answers
- Effective Resume Writing
- HR Interview Questions
- Computer Glossary
- Who is Who
The Problem with Multicollinearity
Introduction
Multicollinearity, a phenomenon characterized by high correlation or linear dependence between predictor variables, poses significant challenges in regression analysis. This article explores the detrimental effects of multicollinearity on statistical models, focusing on issues such as unreliable coefficient estimates, reduced model interpretability, increased standard errors, and inefficient use of variables. We delve into the consequences of multicollinearity and discuss potential solutions to mitigate its impact. By understanding and addressing multicollinearity, researchers, and practitioners can improve the accuracy, reliability, and interpretability of regression models, enabling more robust analysis and informed decision−making.
Problems with Multi−Collinearity
Unreliable coefficient estimates
Because of multicollinearity, determining the unique influence of each predictor variable on the target variable is challenging. The coefficients can become unstable and extremely sensitive to slight changes in the data, resulting in untrustworthy estimations.
Incorrect interpretations of the link between predictor variables and the target variable might result from unreliable coefficient estimations. In the presence of multicollinearity, determining the real impact of each predictor variable becomes difficult since the estimates might be substantially impacted by collinear interactions among the predictors.
To mitigate the issue of unreliable coefficient estimates caused by multicollinearity, it is essential to identify and address the collinear variables. This can involve techniques such as removing one of the correlated variables, transforming the variables, or using regularization methods like ridge regression or lasso regression, which can help stabilize the coefficient estimates and reduce their sensitivity to multicollinearity.
Reduced model interpretability
When predictor variables are highly linked, interpreting the coefficients becomes difficult. The inclusion of multiple associated predictors may obscure or distort the connection between a given predictor and the target variable.
One of the issues in the presence of multicollinearity is limited model interpretability. A strong correlation or linear dependency between predictor variables in a regression model is referred to as multicollinearity. This association might make interpreting the specific impacts of each predictor on the target variable challenging.
The effects of predictor variables get muddled when they are highly linked. Because changes in one predictor may be followed by changes in other linked predictors, it becomes difficult to identify particular impacts to each variable. As a result, the coefficients' meaning becomes less apparent.
For example, in the basic instance of two strongly correlated predictors, determining which predictor is genuinely causing changes in the target variable may be difficult. The coefficient estimates of the associated predictors may become unstable, with surprising signs and magnitudes.
The effects of predictor variables get muddled when they are highly linked. Because changes in one predictor may be followed by changes in other linked predictors, it becomes difficult to identify particular impacts to each variable. As a result, the coefficients' meaning becomes less apparent.
Techniques such as variance inflation factor (VIF) analysis, correlation analysis, or dimensionality reduction approaches like as principal component analysis (PCA) can be used to minimize the issue of diminished interpretability. These methods aid in the identification of collinear variables and give a better understanding of the interactions between predictors and the target variable.
Increased standard errors
Multicollinearity increases the standard errors of the coefficient estimations. This can result in broader confidence ranges and lower statistical significance, making it more difficult to identify important impacts.
In the context of multicollinearity, growing standard errors of coefficient estimates are one of the problems that develop. Multicollinearity is the term used to describe high correlation or linear dependence between predictor variables in a regression model.
As a result, the calculated coefficients become less dependable, and the standard errors grow. Higher standard errors imply higher uncertainty in the coefficient estimations, which might alter the variables' statistical significance. As a result, the calculated coefficients may have broader confidence ranges and lower t-statistics, making it more difficult to establish if the coefficients deviate substantially from zero.
Increased standard errors can contribute to decreased statistical power since it becomes more difficult to identify meaningful effects of predictors on the target variable. It can also have an impact on model interpretation by making it more difficult to determine the degree and direction of the associations between predictors and the target variable.
Inefficient use of variables:
Multicollinearity in the model denotes redundant information. When variables are highly linked, they may provide comparable information, which can lead to inefficiencies and overfitting.
Another issue that occurs in the setting of multicollinearity is the wasteful usage of variables. High correlation or linear dependency between predictor variables in a regression model is referred to as multicollinearity.
When there is multicollinearity, it means that several predictor variables are delivering duplicate or extremely comparable information. This duplication causes the model's variables to be used inefficiently.
Inefficient variable usage implies that the collinear predictors do not provide unique or independent information to the model. Instead, they capture comparable features of the data, which might result in duplication and overfitting.
Inefficient use of variables can result in several issues:
Increased complexity: By incorporating numerous variables that give identical information, multicollinearity might inject additional complexity into the model. This can make the model more difficult to comprehend and limit its capacity to generalize.
Unreliable coefficient estimations: Multicollinearity can induce instability and sensitivity in coefficient estimates.
Overfitting: When a model is overfitting, it performs well on the training data but struggles to generalize to new, untested data. This can happen when redundant variables are included in the model. Poor forecasting performance and limited model applicability in practical applications might result from overfitting.
The inefficient use of variables in the setting of multicollinearity can be addressed using variable selection techniques (e.g., stepwise regression, lasso regression) or dimensionality reduction approaches (e.g., principal component analysis, factor analysis). These strategies aid in the identification and elimination of unnecessary variables, resulting in a more efficient and parsimonious model.
By addressing multicollinearity and encouraging variable efficiency, the model's complexity may be lowered, the stability of coefficient estimates enhanced, and the danger of overfitting minimized. This allows for a more effective and interpretable model that focuses on the key predictors associated with the target variable.
Ways to Deal with Multicollinearity
Dealing with multicollinearity requires implementing appropriate strategies to address the challenges it poses in regression analysis.
Several approaches can be employed, including:
Variable Selection: Identify and remove redundant variables by using techniques like stepwise regression or lasso regression to select the most relevant predictors.
Dimensionality Reduction: Apply methods such as principal component analysis (PCA) or factor analysis to transform the correlated predictors into a smaller set of uncorrelated variables.
Data Collection: Obtain additional data to increase the variability and reduce the correlation among predictors.
Domain Knowledge: Leverage subject−matter expertise to carefully analyze the variables and determine which ones are most meaningful for the model.
Conclusion
Multicollinearity poses significant challenges in regression analysis, including unreliable coefficient estimates, reduced interpretability, increased standard errors, and inefficient use of variables. Addressing multicollinearity requires employing strategies such as variable selection, dimensionality reduction, data collection, and leveraging domain knowledge. By implementing these approaches, researchers can mitigate the adverse effects of multicollinearity and enhance the accuracy and interpretability of regression models. It is crucial to recognize the presence of multicollinearity, apply appropriate techniques to identify and manage it, and ensure the reliability and effectiveness of regression analysis in making informed decisions based on the relationships between variables.
To Continue Learning Please Login