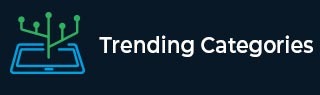
Data Structure
Networking
RDBMS
Operating System
Java
MS Excel
iOS
HTML
CSS
Android
Python
C Programming
C++
C#
MongoDB
MySQL
Javascript
PHP
Physics
Chemistry
Biology
Mathematics
English
Economics
Psychology
Social Studies
Fashion Studies
Legal Studies
- Selected Reading
- UPSC IAS Exams Notes
- Developer's Best Practices
- Questions and Answers
- Effective Resume Writing
- HR Interview Questions
- Computer Glossary
- Who is Who
Purpose of syslog data valuable in Machine learning
Introduction
The amount of data being produced now in the digital age has multiplied tremendously. As a result, companies produce enormous amounts of data every second. Using this information can help businesses run more efficiently, analyse client behaviour, and spot security problems, among other things. It can be difficult to manage and process such a large volume of data, though. Here, machine learning (ML) enters the picture.
Artificial intelligence (AI) in the form of machine learning enables computers to learn from data without explicit programming. It is employed to draw conclusions from data, identify patterns, and create predictions. We will talk about the use of syslog data in machine learning in this article.
Purpose of syslog data valuable
Network hardware like routers, switches, and firewalls produce a particular kind of log data known as syslog. It includes data on user actions, security incidents, and network activity such as system faults. IT administrators depend on syslog data since it aids in monitoring and troubleshooting network issues.
Syslog data, however, can also be useful for machine learning. Using syslog data in machine learning can be done in the following ways −
Network Anomaly Detection
Network anomaly detection is a crucial use of syslog data in machine learning. Anomaly detection is the process of finding out-of-the-ordinary events or patterns in data. Syslog data can be used for network anomaly detection to find unusual network activities like attempts at unauthorized access, breaches, and attacks.
The syslog data can be analyzed with machine learning algorithms to find patterns that point to unusual activities. These examples can then be utilized to prepare a model to distinguish network peculiarities. The model can be utilized to alarm the IT group about potential security breaks, permitting them to make a move before any huge harm happens.
Performance Optimization
Data from syslog can also be used to improve the performance. Execution enhancement alludes to the distinguishing proof of bottlenecks and streamlining of organization execution. Syslog data can be used in network performance optimization to find bottlenecks like slow network response times or high network latency.
Syslog data can be analyzed with machine learning algorithms to find patterns that point to network bottlenecks. By altering network settings, such as increasing bandwidth or changing network routing, the model can be used to improve network performance.
Fraud Detection
Syslog information can likewise be utilized for extortion identification. The process of identifying fraudulent activities, such as identity theft or credit card fraud, is referred to as fraud detection. Syslog data can be used for fraud detection to find patterns that point to fraudulent activities.
AI calculations can be utilized to dissect the syslog information and recognize designs that demonstrate fake exercises. The IT team can be notified of potentially fraudulent activities using the model, allowing them to act before significant damage is done.
Note
For fraud detection, performance optimization, network anomaly detection, and predictive maintenance, syslog data can be useful in the machine learning. AI calculations can break down the syslog information and recognize designs that show strange exercises, hardware disappointment, network bottlenecks, or deceitful exercises. The models prepared on syslog information can assist the IT with joining to make preventive moves before any huge harm happens. As a result, the syslog data should be properly collected and stored so that it can be utilized in the future. Syslog data can be a great source of data for Machine Learning applications.
However, using the syslog data for machine learning presents some difficulties. The substantial volume of data generated by network devices is one of the major obstacles. The infrastructure and resources required to collect and store this data are significant. Syslog data's lack of standardization presents another obstacle. It can be difficult to effectively analyze syslog data because different network devices may generate it in various formats. As a result, the syslog data format must be standardized to guarantee compatibility with various network devices.
Additionally, accurate models are created by Machine Learning algorithms with a large amount of training data. Therefore, the success of Machine Learning applications depends on the quality of the syslog data. Clean, consistent, and pertinent to the use case ought to be the syslog data.
Challenges in ML syslog
While syslog information can be significant for AI applications, there are a few difficulties to survive. It may be challenging to effectively collect, store, and analyze syslog data due to these obstacles. In this part, we will examine a portion of the difficulties related with syslog information and how they can be tended to.
Data Volume
The substantial volume of data generated by network devices is one of the primary obstacles that arise when employing syslog data for machine learning. Network gadgets can create a monstrous measure of syslog information consistently, and gathering and putting away this information can be a test. Putting away huge volumes of syslog information can require critical assets and framework. To deal with the volume of syslog data, a dependable and scalable infrastructure is therefore absolutely necessary.
Information Quality
The nature of syslog information is significant for the progress of AI applications. Notwithstanding, syslog information might contain blunders, irregularities, or superfluous data, which can influence the precision of the AI models. Before using syslog data for machine learning, it is therefore essential to ensure its quality. This can be accomplished by using data cleaning methods like removing duplicate data, fixing errors, and removing irrelevant data.
Data formats
Syslog data formats lack of standardization is another obstacle when using syslog data for machine learning. Syslog data can be difficult to analyze because different network devices might produce it in different formats. Thus, the syslog information design should be normalized to ensure similarity with different organization gadgets. This can be accomplished by collecting syslog data using a standard syslog protocol like RFC 5424.
Security and Privacy
Syslog data may include sensitive data like IP addresses, usernames, and passwords. Syslog data security and privacy must therefore be guaranteed. This can be accomplished by encrypting the syslog data while it is being transmitted and stored, putting in place access controls to restrict who can access the syslog data, and making sure that data privacy laws are followed.
Interpretation of Models Syslog-based machine learning models can be complicated and difficult to understand. This can make it trying to comprehend the bits of knowledge created by AI models and settle on informed choices in view of these experiences. As a result, it is critical to create transparent and comprehensible Machine Learning models. This can be accomplished by involving interpretable AI calculations and giving clarifications to the choices made by the models.
Conclusion
In conclusion, the large amount of data, issues with data quality, a lack of standardization, concerns about data privacy and security, and difficulties with model interpretation make it difficult to use syslog data in Machine Learning applications. Implementing a robust and scalable infrastructure, ensuring the quality and standardization of syslog data, protecting data privacy, and developing transparent and interpretable Machine Learning models are all ways to address these issues. By tending to these difficulties, associations can use syslog information to further develop their organization security, diminish margin time, increment efficiency, and save costs.