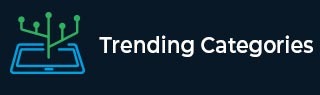
Data Structure
Networking
RDBMS
Operating System
Java
MS Excel
iOS
HTML
CSS
Android
Python
C Programming
C++
C#
MongoDB
MySQL
Javascript
PHP
Physics
Chemistry
Biology
Mathematics
English
Economics
Psychology
Social Studies
Fashion Studies
Legal Studies
- Selected Reading
- UPSC IAS Exams Notes
- Developer's Best Practices
- Questions and Answers
- Effective Resume Writing
- HR Interview Questions
- Computer Glossary
- Who is Who
Role of Big Data, Machine Learning, and Data Science in education
All education sectors are rapidly adapting to the new possibilities of big data, machine learning, and data science. This shift is occurring when the economy is undergoing rapid transformation. Due to recent data collection, processing, and analysis developments, educational institutions know much more about their student's behavior, interests, and academic performance. This is due to the vastly increased resources available to today's classrooms, which is one of the main reasons for the change.
Bio Data in education
The field of education generates a great deal of data that may be put to many different pedagogical purposes. You can research most of these techniques on the web, and information dissemination models are easy to discover in their many manifestations.
There are several resources available to anybody seeking knowledge. Predicting which students will drop out of school is a significant advantage of utilizing big data in education. Teachers might identify students experiencing difficulties in class early on by searching for patterns in the data. If the kid needs further assistance, instructors can provide it after identifying any warning signs. At the moment, this is the finest educational opportunity available.
Moreover, a more individualized education might result from processing massive volumes of data. Teachers may learn a lot about their students' preferred learning styles, strengths, and areas for growth by doing data analysis on the information their students voluntarily provide. Teachers may highlight a student's strengths and areas for improvement.
Machine Learning in Education
These developments have allowed AI to make significant progress in various settings. This may be another interesting subfield to explore. This branch of computer science aims to develop methods by which computers can infer the nature of data without being explicitly programmed with that knowledge. This means that machines can do the work even in the absence of explicit instructions. This is because it may be used to assess student data and tailor recommendations to each individual.
Machine learning is used to facilitate adaptive learning, which increases the likelihood of this happening. Faster processing times allow for more individualized instruction to meet the requirements of each learner, and this allows for the satisfaction of the needs of each unique learner. Data collected from students and analyzed using machine learning techniques may reveal areas where pupils might benefit from further instruction.
This can only be accomplished if the students provide their information. This is achieved by identifying which pupils may benefit from supplemental instruction and then providing that instruction. The data is then utilized to provide each student with relevant and timely suggestions for improving their education and connections to relevant and valuable learning materials. Once data is gathered, it may inform the development of suggestions and references for each student's unique course of study.
Data Science in Education
The field of data science examines the many applications of collected data. Student's academic performance and behavioral patterns may be better understood using facts and science. This information has the potential to raise the bar for education worldwide.
These forecasts are arrived at by comparing historical information with current trends. These forecasts are made by comparing current data with historical data. These forecasts are grounded on the study's analyzed and compiled data findings.
We may evaluate the student's academic progress against expected outcomes to achieve this. Teachers will be better equipped to assist these students in achieving their academic objectives if they can identify them early. Teachers will be better able to assist these students to succeed if they can identify them sooner.
Improved Teaching Methods
Data is essential for any predictive endeavor, including big data, machine learning, and data science. This is because much data is involved in each of these disciplines. Teachers may determine the most successful instruction approaches for the students in their classrooms by reviewing student performance data. This technique may be useful to ensure that educators are using strategies with solid empirical support and proven effectiveness.
Improved Student Retention
Students may be more likely to complete their current coursework if they integrate big data, machine learning, and data science into educational settings. Teachers can predict whether or not a kid will drop out of school by observing their conduct and academic achievement. Applying this knowledge might provide services and resources essential to continuing the student's educational trajectory.
The likelihood of a student dropping out or doing poorly in a course may also be predicted using machine learning algorithms' data on their behavior and academic performance. One way to achieve this would be to analyze the data to determine which pupils have the best grasp of their performance and habits.
Conclusion
If integrated into classrooms, big data, machine learning, and data science may greatly improve students' educational experiences. That would be tremendously useful. To put it briefly, such a shift would be quite encouraging.
Finding at-risk students, tailoring instruction, and raising overall academic performance are all possible outcomes of educators' increasing use of data analytics. Data analytics has various uses, one of which is increasing the effectiveness of these processes.
Predictive analytics helps identify which students need more support to achieve their academic objectives, while machine learning algorithms can provide individualized guidance and assistance to all students.
It is possible to use this technological tool for educational use. By analyzing student information, we can provide actionable insights that may be utilized to enhance the quality of instruction all students receive. Data science is, directly and indirectly, responsible for making this a reality.
This could raise the bar for everything and is now technically feasible. When students grasp these other perspectives, they can better apply what they have learned in the classroom.