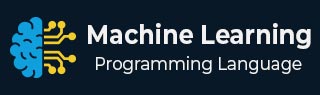
- Machine Learning Basics
- Machine Learning - Home
- Machine Learning - Getting Started
- Machine Learning - Basic Concepts
- Machine Learning - Python Libraries
- Machine Learning - Applications
- Machine Learning - Life Cycle
- Machine Learning - Required Skills
- Machine Learning - Implementation
- Machine Learning - Challenges & Common Issues
- Machine Learning - Limitations
- Machine Learning - Reallife Examples
- Machine Learning - Data Structure
- Machine Learning - Mathematics
- Machine Learning - Artificial Intelligence
- Machine Learning - Neural Networks
- Machine Learning - Deep Learning
- Machine Learning - Getting Datasets
- Machine Learning - Categorical Data
- Machine Learning - Data Loading
- Machine Learning - Data Understanding
- Machine Learning - Data Preparation
- Machine Learning - Models
- Machine Learning - Supervised
- Machine Learning - Unsupervised
- Machine Learning - Semi-supervised
- Machine Learning - Reinforcement
- Machine Learning - Supervised vs. Unsupervised
- Machine Learning Data Visualization
- Machine Learning - Data Visualization
- Machine Learning - Histograms
- Machine Learning - Density Plots
- Machine Learning - Box and Whisker Plots
- Machine Learning - Correlation Matrix Plots
- Machine Learning - Scatter Matrix Plots
- Statistics for Machine Learning
- Machine Learning - Statistics
- Machine Learning - Mean, Median, Mode
- Machine Learning - Standard Deviation
- Machine Learning - Percentiles
- Machine Learning - Data Distribution
- Machine Learning - Skewness and Kurtosis
- Machine Learning - Bias and Variance
- Machine Learning - Hypothesis
- Regression Analysis In ML
- Machine Learning - Regression Analysis
- Machine Learning - Linear Regression
- Machine Learning - Simple Linear Regression
- Machine Learning - Multiple Linear Regression
- Machine Learning - Polynomial Regression
- Classification Algorithms In ML
- Machine Learning - Classification Algorithms
- Machine Learning - Logistic Regression
- Machine Learning - K-Nearest Neighbors (KNN)
- Machine Learning - Naïve Bayes Algorithm
- Machine Learning - Decision Tree Algorithm
- Machine Learning - Support Vector Machine
- Machine Learning - Random Forest
- Machine Learning - Confusion Matrix
- Machine Learning - Stochastic Gradient Descent
- Clustering Algorithms In ML
- Machine Learning - Clustering Algorithms
- Machine Learning - Centroid-Based Clustering
- Machine Learning - K-Means Clustering
- Machine Learning - K-Medoids Clustering
- Machine Learning - Mean-Shift Clustering
- Machine Learning - Hierarchical Clustering
- Machine Learning - Density-Based Clustering
- Machine Learning - DBSCAN Clustering
- Machine Learning - OPTICS Clustering
- Machine Learning - HDBSCAN Clustering
- Machine Learning - BIRCH Clustering
- Machine Learning - Affinity Propagation
- Machine Learning - Distribution-Based Clustering
- Machine Learning - Agglomerative Clustering
- Dimensionality Reduction In ML
- Machine Learning - Dimensionality Reduction
- Machine Learning - Feature Selection
- Machine Learning - Feature Extraction
- Machine Learning - Backward Elimination
- Machine Learning - Forward Feature Construction
- Machine Learning - High Correlation Filter
- Machine Learning - Low Variance Filter
- Machine Learning - Missing Values Ratio
- Machine Learning - Principal Component Analysis
- Machine Learning Miscellaneous
- Machine Learning - Performance Metrics
- Machine Learning - Automatic Workflows
- Machine Learning - Boost Model Performance
- Machine Learning - Gradient Boosting
- Machine Learning - Bootstrap Aggregation (Bagging)
- Machine Learning - Cross Validation
- Machine Learning - AUC-ROC Curve
- Machine Learning - Grid Search
- Machine Learning - Data Scaling
- Machine Learning - Train and Test
- Machine Learning - Association Rules
- Machine Learning - Apriori Algorithm
- Machine Learning - Gaussian Discriminant Analysis
- Machine Learning - Cost Function
- Machine Learning - Bayes Theorem
- Machine Learning - Precision and Recall
- Machine Learning - Adversarial
- Machine Learning - Stacking
- Machine Learning - Epoch
- Machine Learning - Perceptron
- Machine Learning - Regularization
- Machine Learning - Overfitting
- Machine Learning - P-value
- Machine Learning - Entropy
- Machine Learning - MLOps
- Machine Learning - Data Leakage
- Machine Learning - Resources
- Machine Learning - Quick Guide
- Machine Learning - Useful Resources
- Machine Learning - Discussion
Machine Learning - Simple Linear Regression
Simple linear regression is a type of regression analysis in which a single independent variable (also known as a predictor variable) is used to predict the dependent variable. In other words, it models the linear relationship between the dependent variable and a single independent variable.
Python Implementation
Given below is an example that shows how to implement simple linear regression using the Pima-Indian-Diabetes dataset in Python. We will also plot the regression line.
Data Preparation
First, we need to import the Diabetes dataset from scikit-learn and split it into training and testing sets. We will use 80% of the data for training the model and the remaining 20% for testing.
from sklearn.datasets import load_diabetes from sklearn.model_selection import train_test_split # Load the Diabetes dataset diabetes = load_diabetes() # Split the dataset into training and testing sets X_train, X_test, y_train, y_test = train_test_split(diabetes.data[:, 2], diabetes.target, test_size=0.2, random_state=0) # Reshape the input data X_train = X_train.reshape(-1, 1) X_test = X_test.reshape(-1, 1)
Here, we are using the third feature (column) of the dataset, which represents the mean blood pressure, as our independent variable (predictor variable) and the target variable as our dependent variable (response variable).
Model Training
We will use scikit-learn's LinearRegression class to train a simple linear regression model on the training data. The code for this is as follows −
from sklearn.linear_model import LinearRegression # Create a linear regression object lr_model = LinearRegression() # Fit the model on the training data lr_model.fit(X_train, y_train)
Here, X_train represents the input feature (mean blood pressure) of the training data and y_train represents the output variable (target variable).
Model Testing
Once the model is trained, we can use it to make predictions on the test data. The code for this is as follows −
# Make predictions on the test data y_pred = lr_model.predict(X_test)
Here, X_test represents the input feature of the test data and y_pred represents the predicted output variable (target variable).
Model Evaluation
We need to evaluate the performance of the model to determine its accuracy. We will use the mean squared error (MSE) and the coefficient of determination (R^2) as evaluation metrics. The code for this is as follows −
from sklearn.metrics import mean_squared_error, r2_score # Calculate the mean squared error mse = mean_squared_error(y_test, y_pred) # Calculate the coefficient of determination r2 = r2_score(y_test, y_pred) print('Mean Squared Error:', mse) print('Coefficient of Determination:', r2)
Here, y_test represents the actual output variable of the test data.
Plotting the Regression Line
We can also visualize the regression line to see how well it fits the data. The code for this is as follows −
import matplotlib.pyplot as plt # Plot the training data plt.scatter(X_train, y_train, color='gray') # Plot the regression line plt.plot(X_train, lr_model.predict(X_train), color='red', linewidth=2) # Add axis labels plt.xlabel('Mean Blood Pressure') plt.ylabel('Disease Progression') # Show the plot plt.show()
Here, we are using the scatter() function from the matplotlib library to plot the training data points and the plot() function to plot the regression line. The xlabel() and ylabel() functions are used to label the x-axis and y-axis of the plot, respectively. Finally, we use the show() function to display the plot.
Complete Implementation Example
The complete code for implementing simple linear regression in Python is as follows −
from sklearn.datasets import load_diabetes from sklearn.model_selection import train_test_split from sklearn.linear_model import LinearRegression from sklearn.metrics import mean_squared_error, r2_score import matplotlib.pyplot as plt # Load the Diabetes dataset diabetes = load_diabetes() # Split the dataset into training and testing sets X_train, X_test, y_train, y_test = train_test_split(diabetes.data[:, 2], diabetes.target, test_size=0.2, random_state=0) # Reshape the input data X_train = X_train.reshape(-1, 1) X_test = X_test.reshape(-1, 1) # Create a linear regression object lr_model = LinearRegression() # Fit the model on the training data lr_model.fit(X_train, y_train) # Make predictions on the test data y_pred = lr_model.predict(X_test) # Calculate the mean squared error mse = mean_squared_error(y_test, y_pred) # Calculate the coefficient of determination r2 = r2_score(y_test, y_pred) print('Mean Squared Error:', mse) print('Coefficient of Determination:', r2) # Plot the training data plt.figure(figsize=(7.5, 3.5)) plt.scatter(X_train, y_train, color='gray') # Plot the regression line plt.plot(X_train, lr_model.predict(X_train), color='red', linewidth=2) # Add axis labels plt.xlabel('Mean Blood Pressure') plt.ylabel('Disease Progression') # Show the plot plt.show()
Output
On executing this code, you will get the following plot as the output and it will also print the Mean Squared Error and the Coefficient of Determination on the terminal −
Mean Squared Error: 4150.680189329983 Coefficient of Determination: 0.19057346847560164
