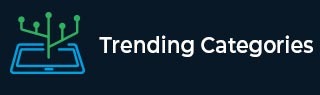
Data Structure
Networking
RDBMS
Operating System
Java
MS Excel
iOS
HTML
CSS
Android
Python
C Programming
C++
C#
MongoDB
MySQL
Javascript
PHP
Physics
Chemistry
Biology
Mathematics
English
Economics
Psychology
Social Studies
Fashion Studies
Legal Studies
- Selected Reading
- UPSC IAS Exams Notes
- Developer's Best Practices
- Questions and Answers
- Effective Resume Writing
- HR Interview Questions
- Computer Glossary
- Who is Who
Future of Machine Learning in Finance
Machine learning is silent, continual progress. Machine Learning (ML) is a branch of AI that analyses data to make predictions, provide suggestions, and enhance performance over time. It does this by training neural networks, which are complicated mathematical structures, on massive datasets. This capability to increase precision and velocity in human decision-making and productivity has the potential to revolutionize the financial services sector and is already doing so. Machine learning has advanced to the point where a new term, "deep learning," has been coined to describe it. In 1930, the first neural networks were presented, designed to mimic the brain's operations. Teaching machines to do tasks for us was the first step towards transforming the world; now, even that is unnecessary.
What is Machine Learning?
In this setting, artificial intelligence can be viewed as an AI-based strategy. It lets robots make judgments based on facts rather than predetermined rules, improving efficiency. Predictions based on past experience are the sole foundation of machine learning. This is how businesses segment their customers and provide individualized service based on their online habits and preferences. These algorithms are crafted in a way that exposes users to fresh data that may be used to refine an organization's approach.
Can Machine Learning Help the Finance Industry?
Some of the most popular applications of machine learning in the financial sector include fraud detection; risk management; process automation; data analytics; client help; and algorithmic trading. These technologies are currently used in more than half of all operational capacities. The field of artificial intelligence in Finance is expanding, with full financial independence as the ultimate aim.
General ledger technology, a close financial solution, and workflow automation are only a few of the areas where Finance has made significant investments in technology, as reported by Gartner's 2022 study of finance executives. It's becoming increasingly apparent that AI and ML will play a significant role in the banking sector of the future. The survey also indicates that by 2025, most CEOs expect to have achieved their goal of a "touchless financial close," in which no human workers are involved in any part of the financial close process.
The Top 10 Applications of Machine Learning in Finance
Predictive models that minimize risk and error can be created using machine learning. In the realm of Finance, new prospects and uses for machine learning are constantly appearing. Some well-established applications of this cutting-edge technology in the banking sector are outlined below.
Automation of the data input, monitoring, and updating process: saving time and effort on mundane routine operations.
Investment portfolio construction and management using computer programs (Robo-advisors).
Trading algorithms allow for rapid pattern recognition and the creation of precise trading strategies.
High-Frequency Trading (HFT) is the process of quickly spotting market opportunities and acting on them.
Identifying fraudulent actions such as insider trading and money laundering is known as fraud detection.
Credit scoring, underwriting, and loans; the process of determining a borrower's creditworthiness. Also, Building chatbots for use in areas such as customer service or investment advice.
To handle hazards, one must first be able to recognize them. Settlements in international trade: computerizing the payments procedure.
Analysis of documents and other forms of unstructured data, such as financial reports and contracts.
The process of acquiring and onboarding new customers can be automated, improving the customer experience by tailoring services and making specific suggestions.
Benefits of Financial Applications in machine learning
Among the many advantages of using machine learning in the financial sector are those listed below.
Reducing the Impact of Human Error
In the financial industry, even a minor mistake can have far-reaching consequences, including the loss of millions of dollars. Mistakes made by humans occur constantly. Machine learning algorithms can replace or supplement human workers to greatly improve data accuracy.
Economical
The state of the art in AI and ML has evolved throughout time. It may be worthwhile in the long term despite the initial outlay and ongoing expenses.
It saves time
The use of machine learning allows for the efficient and speedy resolution of problems. It can streamline time-consuming manual tasks, aid in the formation of nuanced judgments, and produce reliable forecasts.
Objective
The banking sector desperately needs machine learning to make such impartial and open-source decisions. When it comes to data selection and making decisions, machine learning algorithms are often more rational than human judgment.
Workload Lightening
Machine learning is a time and labor saver since it can do complex, repetitive jobs.
Careers of Machine Learning in the financial industry
There is a growing need for skilled professionals with experience in machine learning, which is still a relatively new concept in banking and other fields. Jobs in machine learning can be found in many different industries, including IT, software development, and economics. Machine learning is significant since it provides a fresh viewpoint on consumer tendencies and organizational habits. According to the BLS, most people working in the sector are classified as computer and information research analysts. It's helpful in automating the processes that would otherwise be handled by hand, and it can help drive corporate results like cost savings and new ways of working.
Decisions in the banking industry are increasingly being informed by data. Experts in machine learning will find increasing demand for their services in the financial industry as the subject develops. Today, many large corporations use machine learning, like Facebook, Uber, Ola, Google, etc. Opportunities abound in the financial sector for anyone with machine learning expertise. There is a high need for machine learning professionals in the banking industry, and they may expect to earn above-average salaries as a result.
Data analyst in machine learning: $131,490 annually
Quantitative research analyst: $119,222.
Engineer in machine learning: $129,101
Statistical modeler: $142,379
Financial data scientist: $117,527.
Qualifications needed to apply for positions in machine learning
Positions in machine learning can range widely and call for a wide range of expertise and experience. Strong technical and programming abilities are required of a machine-learning engineer, whereas strong mathematics and statistical skills are required of a machine-learning scientist. Common requirements for those seeking employment in machine learning include the following:
A baccalaureate degree in computer science or a closely related discipline
Knowledge of statistical methods and computer-learning routines
Capacity for handling massive datasets
Competence in using a variety of programming languages
Capacity to explain data analysis findings to others who aren't experts in the field
Conclusion
The future of machine learning in banking, its potential applications, and the jobs it could create are all worth exploring. Many new machine learning finance positions have emerged as a result of ML's wide range of financial applications.