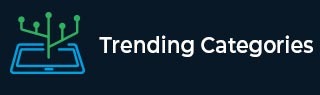
Data Structure
Networking
RDBMS
Operating System
Java
MS Excel
iOS
HTML
CSS
Android
Python
C Programming
C++
C#
MongoDB
MySQL
Javascript
PHP
Physics
Chemistry
Biology
Mathematics
English
Economics
Psychology
Social Studies
Fashion Studies
Legal Studies
- Selected Reading
- UPSC IAS Exams Notes
- Developer's Best Practices
- Questions and Answers
- Effective Resume Writing
- HR Interview Questions
- Computer Glossary
- Who is Who
What are the methods of outlier detection?
There are various methods of outlier detection is as follows −
Supervised Methods − Supervised methods model data normality and abnormality. Domain professionals tests and label a sample of the basic data. Outlier detection can be modeled as a classification issue. The service is to understand a classifier that can identify outliers.
The sample can be used for training and testing. In various applications, the professionals can label only the normal objects, and several objects not connecting the model of normal objects are documented as outliers. There are different methods model the outliers and consider objects not connecting the model of outliers as normal.
Unsupervised Methods − In various application methods, objects labeled as “normal” or “outlier” are not applicable. Therefore, an unsupervised learning approach has to be used. Unsupervised outlier detection methods create an implicit assumption such as the normal objects are considerably “clustered.”
An unsupervised outlier detection method predict that normal objects follow a pattern far more generally than outliers. Normal objects do not have to decline into one team sharing large similarity. Instead, they can form several groups, where each group has multiple features.
This assumption cannot be true sometime. The normal objects do not send some strong patterns. Rather than, they are uniformly distributed. The collective outliers, share large similarity in a small area.
Unsupervised methods cannot identify such outliers efficiently. In some applications, normal objects are separately distributed, and several objects do not follow strong patterns. For example, in some intrusion detection and computer virus detection issues, normal activities are distinct and some do not decline into high-quality clusters.
Some clustering methods can be adapted to facilitate as unsupervised outlier detection methods. The main idea is to discover clusters first, and therefore the data objects not belonging to some cluster are identified as outliers. However, such methods deteriorate from two issues. First, a data object not belonging to some cluster can be noise rather than an outlier. Second, it is expensive to discover clusters first and then discover outliers.
Semi-Supervised Methods − In several applications, although obtaining some labeled instance is possible, the number of such labeled instances is small. It can encounter cases where only a small group of the normal and outlier objects are labeled, but some data are unlabeled. Semi-supervised outlier detection methods were produced to tackle such methods.
Semi-supervised outlier detection methods can be concerned as applications of semisupervised learning approaches. For example, when some labeled normal objects are accessible, it can use them with unlabeled objects that are nearby, to train a model for normal objects. The model of normal objects is used to identify outliers—those objects not suitable the model of normal objects are defined as outliers.