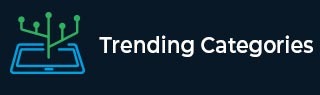
Data Structure
Networking
RDBMS
Operating System
Java
MS Excel
iOS
HTML
CSS
Android
Python
C Programming
C++
C#
MongoDB
MySQL
Javascript
PHP
Physics
Chemistry
Biology
Mathematics
English
Economics
Psychology
Social Studies
Fashion Studies
Legal Studies
- Selected Reading
- UPSC IAS Exams Notes
- Developer's Best Practices
- Questions and Answers
- Effective Resume Writing
- HR Interview Questions
- Computer Glossary
- Who is Who
What about using statistical techniques for spatial data mining?
Statistical spatial data analysis has been a famous techniques to exploring spatial data and analysing geographic data. The term geostatistics is related to continuous geographic area, whereas the term spatial statistics is related to discrete space.
In a statistical model that manages non-spatial records, one generally consider statistical independence between different areas of data. However, different from traditional data sets, there is no such independence among spatially distributed data because in reality, spatial objects are often interrelated, or more exactly spatially colocated, in the sense that the closer the two objects are placed, the more possible they share same properties.
For instance, nature resource, climate, temperature, and economic situations are to be same in geographically closely located areas. Persons even treated this as the first law of geography − Everything is associated to everything else, but nearby things are more associated than isolated things.
Such a feature of close interdependency across adjacent space leads to the concept of spatial autocorrelation. It depends on this concept, spatial statistical modeling methods have been produced with better success.
Spatial data mining will develop spatial statistical analysis techniques and improve them for large amounts of spatial data, with more emphasis on effectiveness, scalability, cooperation with database and data warehouse systems, enhanced customer interaction, and the find of new techniques of knowledge.
A spatial database saves a huge amount of space-related data, including maps, preprocessed remote sensing or medical imaging records, and VLSI chip design data.
Spatial databases have several features that distinguish them from relational databases. They carry topological and distance data, generally organized by sophisticated, multidimensional spatial indexing architecture that are created by spatial data access methods and require spatial reasoning, geometric calculation, and spatial knowledge representation methods.
Spatial data mining defines the extraction of knowledge, spatial relationships, or other interesting designs not explicitly saved in spatial databases. Such mining demands the unification of data mining with spatial database technologies. It can be used for learning spatial records, discovering spatial relationships and relationships among spatial and non-spatial records, constructing spatial knowledge bases, reorganizing spatial databases, and optimizing spatial queries.
A central challenge to spatial data mining is the exploration of efficient spatial data mining techniques because of the large amount of spatial data and the difficulty of spatial data types and spatial access methods. Statistical spatial data analysis has been a famous techniques to exploring spatial data and analysing geographic data.
The term geostatistics is related to continuous geographic space, whereas the spatial statistics is related to discrete space. In a statistical model that manages non-spatial records, one generally consider statistical independence among different areas of data.