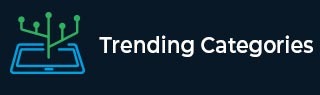
Data Structure
Networking
RDBMS
Operating System
Java
MS Excel
iOS
HTML
CSS
Android
Python
C Programming
C++
C#
MongoDB
MySQL
Javascript
PHP
Physics
Chemistry
Biology
Mathematics
English
Economics
Psychology
Social Studies
Fashion Studies
Legal Studies
- Selected Reading
- UPSC IAS Exams Notes
- Developer's Best Practices
- Questions and Answers
- Effective Resume Writing
- HR Interview Questions
- Computer Glossary
- Who is Who
What are the data Mining methods for Recommender Systems?
Recommender systems can use a content-based approach, a collaborative approach, or a hybrid approach that combines both content-based and collaborative methods.
Content-based − In the content-based approach recommends items that are same to items the customer preferred or queried in the previous. It depends on product features and textual item definition.
In content-based methods, it is calculated based on the utilities assigned by the similar user to different items that are same. Many systems target on recommending items including textual data, including websites, articles, and news messages. They view for commonalities between items. For movies, they can view for same genres, directors, or actors.
For articles, they can view for same terms. Content-based approaches are rooted in data theory. They create use of keywords (defining the items) and customer profiles that include data about users’ tastes and requirements. Such profiles can be obtained explicitly or understand from customer transactional behavior over time.
Collaborative − In the collaborative approach, it can consider a user’s social environment. It recommends items depends on the opinions of different customers who have same tastes or preferences as the user.
Recommender systems need a wide range of methods from information retrieval, statistics, machine learning, and data mining to search for similarities between items and user preferences.
The benefit of recommender systems is that they support personalization for users of e-commerce, developmental one-to-one marketing. Amazon, a pioneer in the need of collaborative recommender systems, provides “a personalized store for each customer” as an element of their marketing strategy.
Personalization can benefit both users and the company included. By receiving more efficient models of their users, companies gain a better understanding of users needs. Serving these needs can result in higher success concerning cross-selling of associated products, upselling, product affinities, one-to-one promotions, higher baskets, and user retention.
A collaborative recommender system attempts to forecast the utility of items for a user, u, depends on items previously rated by different users who are same to u. For instance, when recommending books, a collaborative recommender system tries to discover multiple users who have a history of agreeing with u. Collaborative recommender systems can be memory (or heuristic) based or model based.
Memory-based methods use heuristics to create rating predictions based on the whole set of items previously rated by users. The anonymous rating of an item–user combination can be computed as an aggregate of ratings of the same users for the similar item.