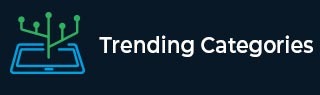
Data Structure
Networking
RDBMS
Operating System
Java
MS Excel
iOS
HTML
CSS
Android
Python
C Programming
C++
C#
MongoDB
MySQL
Javascript
PHP
Physics
Chemistry
Biology
Mathematics
English
Economics
Psychology
Social Studies
Fashion Studies
Legal Studies
- Selected Reading
- UPSC IAS Exams Notes
- Developer's Best Practices
- Questions and Answers
- Effective Resume Writing
- HR Interview Questions
- Computer Glossary
- Who is Who
What is the Role of ML in IoT?
The IoT or Internet of Things is a network of various devices. These devices are interconnected with each other. The devices include vehicles, buildings & other objects embedded with sensors, software & connectivity. Machine learning (ML) is a part of artificial intelligence (AI). The AIs must be trained for them to recognize patterns and make predictions from data. Everything is carried out without any explicit programming. Machine learning has become increasingly important in the creation of IoT applications in recent years.
We will examine machine learning's function in the Internet of Things in this article.
Role of Machine Learning in IoT
Predictive Maintenance
Predictive maintenance involves performing maintenance to stop problems before they happen and making predictions about when machines will break based on data gathered from sensors. Algorithms for machine learning can be taught to analyze sensor data & spot trends that point out when a machine is likely to break down. Reduced downtime, increased safety & lower maintenance costs are all benefits of predictive maintenance.
Anomaly Detection
Anomaly detection involves identifying events or data points that are outside the expected range. In IoT applications, anomaly detection can be used to detect abnormal behavior or malfunctions in devices. Machine learning algorithms can be trained to detect anomalies in sensor data & trigger alerts or actions when anomalies are detected. Anomaly detection can help improve the reliability and safety of IoT devices.
Personalization
Based on user behavior and preferences, machine learning can be used to customize IoT apps. For instance, machine learning algorithms can be used to analyze data from smart homes to identify resident preferences and then adjust the temperature, lighting, and music settings to fit those tastes. Personalization can improve user experience and increase IoT device adoption.
Environmental Monitoring
Data from sensors estimate environmental factors. It measures factors like temperature, humidity & air quality. The environmental conditions in buildings, factories, and other facilities can be monitored and optimized using this data. Based on past data, machine learning can also be used to forecast environmental conditions and modify settings to improve conditions.
Resource Optimization
In IoT applications, machine learning algorithms can be used to maximize the usage of resources like water, electricity, and materials. For example, smart grid sensor data analysis using machine learning algorithms can be used to estimate energy demand and adjust energy production and distribution to maximize efficiency. Costs can be cut and sustainability can be raised with the aid of resource optimization.
Smart Transportation
By forecasting traffic patterns, enhancing routes, and controlling traffic flow, machine learning algorithms can be utilized to optimize transportation systems. To identify anomalies and anticipate maintenance requirements, machine learning can also be used to analyze data from vehicle sensors. Smart mobility may ease congested roads, increase safety, and lower pollution.
Challenges in Using Machine Learning in IoT
IoT applications can greatly benefit from machine learning, but several issues need to be resolved. Some of the challenges include −
Data Quality − Machine learning algorithms require high-quality data to provide accurate predictions. It may be challenging to develop precise machine learning models for IoT applications due to the possibility that the data is noisy, lacking, or inconsistent.
Security − IoT devices could be exposed to security risks like viruses or hacking. Machine learning algorithms can also be vulnerable to attacks such as adversarial examples or model poisoning, which can compromise the accuracy of the model.
Scalability − IoT applications involve large amounts of data & a large number of devices, which can make it difficult to scale machine learning algorithms.
Latency − In many IoT applications, real-time or near-real-time decision-making is crucial. However, machine learning algorithms can require significant computation time, which can introduce latency in decision-making. This can be a critical issue in applications such as autonomous vehicles or industrial automation where rapid response times are essential.
Interoperability − Machine learning algorithms may be challenging to integrate into IoT devices and systems since they are frequently created using various technologies and standards. The development of precise machine-learning models can be challenging due to interoperability constraints, which can include problems with data format, data access, and network connectivity.
Energy Efficiency − IoT devices often have limited power and processing resources, which can make it difficult to run complex machine learning algorithms. The energy required to transmit data to a central processing unit or the cloud for analysis can also be a significant challenge. Energy-efficient machine learning algorithms are necessary to overcome these challenges and ensure that IoT devices can operate within their power and processing limitations.
Conclusion
Machine learning has become an essential tool for developing IoT applications. Machine learning algorithms can be used for predictive maintenance, anomaly detection, personalization, environmental monitoring, resource optimization & smart transportation. However, other issues—like data quality, security & scalability—need to be resolved. However, the potential advantages of machine learning in IoT applications are significant and will keep this industry innovative.
As such, organizations need to invest in developing machine learning capabilities to maximize the potential of IoT. With the proper integration of machine learning and IoT, organizations can optimize operations, enhance user experience, and improve sustainability, among other benefits.
To Continue Learning Please Login