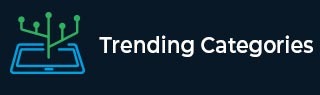
Data Structure
Networking
RDBMS
Operating System
Java
MS Excel
iOS
HTML
CSS
Android
Python
C Programming
C++
C#
MongoDB
MySQL
Javascript
PHP
Physics
Chemistry
Biology
Mathematics
English
Economics
Psychology
Social Studies
Fashion Studies
Legal Studies
- Selected Reading
- UPSC IAS Exams Notes
- Developer's Best Practices
- Questions and Answers
- Effective Resume Writing
- HR Interview Questions
- Computer Glossary
- Who is Who
What are the applications of Memory Based Reasoning?
The human ability to reason from experience based on the ability to identify suitable examples from the prior. A doctor diagnosing infection, a claims analyst flagging fraudulent insurance property, and a mushroom hunter pointing Morels are following a same procedure.
Each first recognizes same cases from experience and then uses what their knowledge of those methods to the issues at hand. This is the importance of memory-based reasoning. A database of known data is searched to discover preclassified records same to a new data. These neighbors are used for classification and computation.
There are various applications of Memory Based Reasoning which are as follows −
Fraud detection − New cases of fraud are same to be known cases. MBR can discover and flag them for more investigation.
Customer response prediction − The next customers probable to respond to an offer are probably same to prior customers who have acknowledged. MBR can simply recognize the next likely customers.
Medical treatments − The efficient treatment for a given patient is possible the treatment that resulted in the best results for same patients. MBR can discover the treatment that makes the best results.
Classifying responses − Free-text responses, including those on the U.S. Census form for occupation and market or complaints appearing from users, required to be classified into a fixed set of codes. MBR can process the free-text and creates the codes.
The strengths of MBR is its ability to use information. Unlike several data mining methods, it does not care about the structure of the data. It only cares about the continuation of two operations: A distance function able of computing a distance between some two data and a combination function capable of merging results from multiple neighbors to appear at an answer.
These functions are defined for several types of records, such as records with complex or unusual data types including geographic areas, images, and free text that are generally complex to manage with other analysis techniques.
The second strength of MBR is its ability to modify. Merely incorporating new record into the historical database creates it applicable for MBR to understand about new elements and new definitions of previous ones. MBR also makes best results without a long time devoted to training or to massaging incoming information into the right format.
These benefits appear at a cost. MBR influence to be a resource hog because a large amount of historical record should be readily available for discovering neighbors. Classifying new data can require processing all the historical data to discover the most similar neighbors—a more slow process than using an already-trained neural network or an already-constructed decision tree. There is also the difficult of discovering good distance and combination functions, which needed a bit of trial and error and intuition.
To Continue Learning Please Login