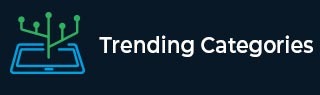
Data Structure
Networking
RDBMS
Operating System
Java
MS Excel
iOS
HTML
CSS
Android
Python
C Programming
C++
C#
MongoDB
MySQL
Javascript
PHP
Physics
Chemistry
Biology
Mathematics
English
Economics
Psychology
Social Studies
Fashion Studies
Legal Studies
- Selected Reading
- UPSC IAS Exams Notes
- Developer's Best Practices
- Questions and Answers
- Effective Resume Writing
- HR Interview Questions
- Computer Glossary
- Who is Who
Found 427 Articles for Data Mining

497 Views
GSP stands for Generalised Sequential Patterns. It is a sequential pattern mining method that was produced by Srikant and Agrawal in 1996. It is an expansion of their seminal algorithm for usual itemset mining, referred to as Apriori. GSP needs the downward-closure natures of sequential patterns and adopts a several-pass, students create-and-test approach.The algorithm is as follows. In the first scan of the database, it can discover some frequent items, i.e., those with minimum support. Each item yields a 1-event frequent sequence including that item. Each subsequent pass begins with a seed group of sequential patterns and the group of ... Read More

9K+ Views
Sequential pattern mining is the mining of frequently appearing series events or subsequences as patterns. An instance of a sequential pattern is users who purchase a Canon digital camera are to purchase an HP color printer within a month.For retail information, sequential patterns are beneficial for shelf placement and promotions. This industry, and telecommunications and different businesses, can also use sequential patterns for targeted marketing, user retention, and several tasks.There are several areas in which sequential patterns can be used such as Web access pattern analysis, weather prediction, production processes, and web intrusion detection.Given a set of sequences, where each ... Read More

270 Views
STREAM is an individual-pass, constant element approximation algorithm that was produced for the k-medians problem. The k-medians problem is to cluster N data points into k clusters or groups such that the sum squared error (SSQ) between the points and the cluster center to which they are assigned is minimized. The idea is to assign similar points to the same cluster, where these points are dissimilar from points in other clusters.In the stream data model, data points can only be seen once, and memory and time are limited. It can implement high-quality clustering, the STREAM algorithm processes data streams in ... Read More

790 Views
Data stream clustering is described as the clustering of data that appar continuously including telephone data, multimedia data, monetary transactions etc. Data stream clustering is generally treated as a streaming algorithm and the objective is, given a sequence of points, to make a best clustering of the stream, utilizing a small amount of memory and time.Some applications needed the automated clustering of such data into set based on their similarities. Examples contains applications for web intrusion detection, analyzing Web clickstreams, and stock market analysis.There are several dynamic methods for clustering static data sets clustering data streams places additional force on ... Read More

595 Views
A user supports two input parameters including the min support threshold, σ, and the error bound previously, indicated as ε. The incoming stream is theoretically divided into buckets of width w = [1/ε].Let N be the current stream length, i.e., the number of items view so far. The algorithm needs a frequency-list data structure for all elements with frequency higher than 0. For every item, the list supports f, the approximate frequency count, and ∆, the maximum possible error of f.The algorithm procedure buckets of items as follows. When a new bucket arrives in, the items in the bucket are ... Read More

2K+ Views
Randomized Algorithms − Randomized algorithms in the form of random sampling and blueprint, are used to deal with large, high-dimensional data streams. The need of randomization leads to simpler and more effective algorithms in contrast to known deterministic algorithms.If a randomized algorithm continually returns the correct answer but the running times change, it is called a Las Vegas algorithm. In contrast, a Monte Carlo algorithm has bounds on the running time but cannot restore the true result. It can usually consider Monte Carlo algorithms. The importance of a randomized algorithm is simply as a probability distribution over a group of ... Read More

251 Views
The sequential exception technique simulates the method in which humans can distinguish unusual sets from between a sequence of supposedly like objects. It helps implicit redundancy of the data.Given a data set, D, of n objects, it construct a sequence of subsets, {D1, D2, ..., Dm}, of these objects with 2 ≤ m ≤ n including$$\mathrm{D_{j−1}\subset D_{j}\:\:where\: D_{j}\subseteq D}$$Dissimilarities are assessed between subsets in the series. The technique learns the following terms which are as follows −Exception set − This is the set of deviations or outliers. It is defined as the smallest subset of objects whose removal results in ... Read More

112 Views
A partitioning clustering method is desirable because it minimizes the distance among sets and their cluster centers. If it can choose the k-means method, a cluster center cannot be available given the existence of obstacles.For instance, the cluster can turn out to be in the center of a lake. In other words, the k-medoids method chooses an object inside the cluster as a center and thus guarantees that a problem cannot appear.At each time a new medoid is selected, the distance among each object and its newly selected cluster center has to be recalculated. Because there can be obstacles among ... Read More

3K+ Views
PROCLUS stands for Projected Clustering. It is a usual dimension-reduction subspace clustering techniques. That is, rather than starting from individual-dimensional spaces, it begins by finding an original approximation of the clusters in the high-dimensional attribute area.Each dimension is created a weight for each cluster, and the refreshed weights are used in the next iteration to recreate the clusters. This leads to the exploration of dense areas in all subspaces of some convenient dimensionality and prevents the generation of a huge number of overlapped clusters in projected dimensions of lower dimensionality.PROCLUS discover the best group of medoids by a hill-climbing phase ... Read More

2K+ Views
CLIQUE was the first algorithm projected for dimension-growth subarea clustering in high-dimensional area. In dimension-growth subarea clustering, the clustering process begins at single-dimensional subspaces and increase upward to higher-dimensional ones.Because CLIQUE partitions each dimension such as grid architecture and decides whether a cell is dense based on the multiple points it includes. It can be looked as an integration of density-based and grid-based clustering approaches.The ideas of the CLIQUE clustering algorithm are as follows −Given a large group of multidimensional data points, the data area is generally not uniformly engaged by the data points. CLIQUE’s clustering recognizes the sparse and ... Read More