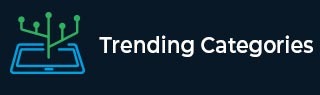
Data Structure
Networking
RDBMS
Operating System
Java
MS Excel
iOS
HTML
CSS
Android
Python
C Programming
C++
C#
MongoDB
MySQL
Javascript
PHP
Physics
Chemistry
Biology
Mathematics
English
Economics
Psychology
Social Studies
Fashion Studies
Legal Studies
- Selected Reading
- UPSC IAS Exams Notes
- Developer's Best Practices
- Questions and Answers
- Effective Resume Writing
- HR Interview Questions
- Computer Glossary
- Who is Who
How Does Netflix Use Machine Learning?
With a vast library of movies, TV shows, and documentaries, Netflix faces the challenge of helping users discover relevant content from a wide array of options. To address this challenge, machine learning algorithms step in as vital components of the process, carefully examining user behavior, preferences, and historical data to provide accurate recommendations.
In this article, we will explore how Netflix effectively utilizes machine learning across its operations.
Machine Learning Applications in Netflix
Below are some Applications of machine learning in Netflix−
Content Recommendation System
Netflix's content recommendation system is one of its most prominent machine-learning applications. By analyzing user viewing history, ratings, and interactions, Netflix's algorithms generate personalized recommendations tailored to each individual's taste. This helps users discover new content that aligns with their preferences, increasing user engagement and satisfaction.
Personalized User Experience
Machine learning allows Netflix to provide a personalized user experience by dynamically adapting its interface based on individual preferences. By analyzing factors such as language preferences, genre preferences, and viewing habits, Netflix can customize the platform's layout, artwork, and content categories, ensuring a seamless and tailored experience for each user.
Quality Control and Content Analysis
Maintaining a high standard of content quality is vital for Netflix's success. Machine learning algorithms help automate content analysis by examining various attributes of videos, such as visual and audio quality, genre classification, and content tags. This enables Netflix to identify and rectify any potential issues promptly.
Marketing and User Acquisition
Machine learning also plays a significant role in Netflix's marketing and user acquisition strategies. By analyzing user data, Netflix can target specific audiences with personalized advertising campaigns, optimizing customer acquisition efforts. Machine learning algorithms also help predict user churn, allowing Netflix to implement retention strategies and reduce customer attrition.
Netflix's Recommendation System
Netflix owes much of its success to its highly effective recommendation system. The platform employs a sophisticated blend of collaborative filtering, content-based filtering, and hybrid filtering techniques to deliver precise and relevant recommendations to its users.
Collaborative filtering plays a vital role by examining user behavior and interactions to identify patterns of similarity between individuals. By recognizing shared preferences among users with similar tastes, Netflix suggests content that aligns with their interests. This collaborative approach expands users' viewing choices by introducing them to content enjoyed by others who share their preferences.
Content-based filtering, on the other hand, focuses on the intrinsic qualities of the content itself. By analyzing metadata such as genre, cast, director, and keywords, this method suggests similar content based on the user's previous preferences. In this way, Netflix recommends movies and TV shows that possess similar characteristics to the content the user has enjoyed in the past.
To further enhance the accuracy of its recommendations, Netflix employs a hybrid filtering approach. This technique combines the strengths of collaborative and content-based filtering, leveraging both user behavior and content attributes. By integrating these two perspectives, the hybrid filtering method generates highly personalized recommendations that accurately reflect the user's unique preferences.
Enhancing User Experience with Machine Learning
In addition to recommendations, Netflix employs machine learning to enhance user experience in various other ways.
Dynamic Thumbnails
Netflix leverages the power of machine learning to create captivating and ever-changing thumbnails that truly reflect the essence of each movie or TV show. Through careful analysis of visual elements and user preferences, the platform intelligently selects these thumbnails to grab users' attention and encourage them to click and delve into the content. This smart optimization not only enhances user engagement but also significantly boosts the likelihood of discovering exciting new movies and shows.
Video Encoding Optimization
Efficient video encoding is essential for seamless streaming experiences. Machine learning helps Netflix optimize video encoding parameters based on network conditions, device capabilities, and user preferences. By dynamically adjusting the encoding settings, Netflix ensures that videos are streamed smoothly without compromising quality.
User Interface Optimization
Netflix's user interface is designed to be intuitive and user-friendly. Machine learning algorithms analyze user interactions, such as scrolling behavior, menu choices, and search queries, to continuously optimize the platform's user interface. This iterative process ensures that Netflix's interface remains responsive, intuitive, and easy to navigate.
Ensuring Content Quality and Analysis
To maintain high-quality content and provide an optimal viewing experience, Netflix relies on machine learning algorithms for content quality control and analysis.
Automated Content Tagging
With an extensive library of content, manually tagging each video is a laborious task. Machine learning algorithms automate this process by analyzing video metadata, subtitles, and audio tracks. This allows Netflix to efficiently categorize and tag content, improving searchability and content discovery for users.
Video Quality Control
Machine learning algorithms help Netflix maintain consistent video quality across its platform. By analyzing video metrics, such as resolution, bitrate, and playback performance, Netflix can identify and rectify any potential issues, ensuring that users enjoy a seamless streaming experience.
Predictive Analytics
Netflix utilizes predictive analytics to anticipate user behavior and preferences. By analyzing historical data, user interactions, and external factors, machine learning algorithms predict trends, audience interests, and content demand. This enables Netflix to make data-driven decisions regarding content acquisition, production, and distribution.
Marketing and User Acquisition Strategies
Machine learning has proven invaluable in Netflix's marketing and user acquisition efforts.
Targeted Advertising
Netflix leverages machine learning to optimize targeted advertising campaigns. By analyzing user demographics, viewing habits, and preferences, Netflix can tailor advertisements to specific audiences, increasing the likelihood of conversions and user acquisition.
Customer Segmentation
Machine learning algorithms help Netflix segment its customer base based on various criteria, such as viewing patterns, engagement levels, and preferences. This segmentation allows Netflix to create targeted marketing campaigns that resonate with different user segments, enhancing customer acquisition and retention.
Churn Prediction
Identifying users at risk of churn is essential for reducing customer attrition. Netflix employs machine learning algorithms to predict churn by analyzing user behavior, viewing patterns, and engagement metrics. This allows Netflix to implement proactive strategies, such as personalized recommendations or retention offers, to retain customers and increase loyalty.
The Benefits of Machine Learning for Netflix
The integration of machine learning into Netflix's operations offers several benefits.
Personalization and Customer Satisfaction
By leveraging machine learning algorithms, Netflix can provide highly personalized experiences to its users. Recommendations tailored to individual preferences enhance customer satisfaction and engagement, increasing the likelihood of users continuing their subscriptions and recommending Netflix to others.
Improved Content Discovery
Machine learning enables Netflix to overcome the challenge of content overload. Through accurate recommendations and content categorization, users can easily discover content that aligns with their interests, leading to increased content consumption and user engagement.
Operational Efficiency
Automating various processes, such as content tagging, video quality control, and targeted advertising, streamlines Netflix's operations and improves efficiency. Machine learning algorithms handle these tasks more efficiently than manual approaches, freeing up resources and allowing Netflix to focus on delivering high-quality content and user experiences.
Challenges and Limitations
While machine learning brings significant advantages to Netflix, there are also challenges and limitations to consider.
Privacy and Data Security
Netflix collects vast amounts of user data to train its machine-learning algorithms. Ensuring the privacy and security of user data is crucial to maintain user trust. Netflix employs robust data protection measures and adheres to privacy regulations to safeguard user information.
Bias and Fairness
Machine learning algorithms can inadvertently perpetuate biases if not carefully monitored. Netflix takes steps to mitigate bias in its recommendations and algorithms, striving to provide fair and diverse content suggestions to its users.
Data Scalability
As Netflix's user base continues to grow, scalability becomes a challenge. Processing and analyzing massive amounts of data in real time require scalable machine learning infrastructure and algorithms. Netflix invests in cutting-edge technologies and architectures to handle the scale of its data effectively.
Conclusion
In conclusion, Netflix's success as a streaming giant owes much to the strategic utilization of machine learning. By harnessing the power of advanced algorithms, Netflix provides personalized recommendations, enhances user experience, maintains content quality, and optimizes its marketing strategies. Through ongoing advancements in machine learning, Netflix aims to continue delighting its users with engaging content and seamless viewing experiences.