Practical Deep Learning at Scale with MLflow
Practical Deep Learning at Scale with MLflow
Language - English
Updated on Jan, 2023
About the Book
Book description
Train, test, run, track, store, tune, deploy, and explain provenance-aware deep learning models and pipelines at scale with reproducibility using MLflow
Key Features
- Focus on deep learning models and MLflow to develop practical business AI solutions at scale
- Ship deep learning pipelines from experimentation to production with provenance tracking
- Learn to train, run, tune and deploy deep learning pipelines with explainability and reproducibility
Book Description
The book starts with an overview of the deep learning (DL) life cycle and the emerging Machine Learning Ops (MLOps) field, providing a clear picture of the four pillars of deep learning: data, model, code, and explainability and the role of MLflow in these areas.
From there onward, it guides you step by step in understanding the concept of MLflow experiments and usage patterns, using MLflow as a unified framework to track DL data, code and pipelines, models, parameters, and metrics at scale. You’ll also tackle running DL pipelines in a distributed execution environment with reproducibility and provenance tracking, and tuning DL models through hyperparameter optimization (HPO) with Ray Tune, Optuna, and HyperBand. As you progress, you’ll learn how to build a multi-step DL inference pipeline with preprocessing and postprocessing steps, deploy a DL inference pipeline for production using Ray Serve and AWS SageMaker, and finally create a DL explanation as a service (EaaS) using the popular Shapley Additive Explanations (SHAP) toolbox.
By the end of this book, you’ll have built the foundation and gained the hands-on experience you need to develop a DL pipeline solution from initial offline experimentation to final deployment and production, all within a reproducible and open source framework.
What you will learn
- Understand MLOps and deep learning life cycle development
- Track deep learning models, code, data, parameters, and metrics
- Build, deploy, and run deep learning model pipelines anywhere
- Run hyperparameter optimization at scale to tune deep learning models
- Build production-grade multi-step deep learning inference pipelines
- Implement scalable deep learning explainability as a service
- Deploy deep learning batch and streaming inference services
- Ship practical NLP solutions from experimentation to production
Who this book is for
This book is for machine learning practitioners including data scientists, data engineers, ML engineers, and scientists who want to build scalable full life cycle deep learning pipelines with reproducibility and provenance tracking using MLflow. A basic understanding of data science and machine learning is necessary to grasp the concepts presented in this book.

eBook Preview
Author Details

Packt Publishing
Our students work
with the Best
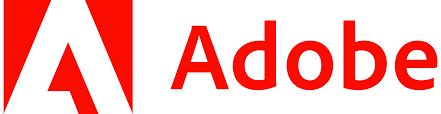
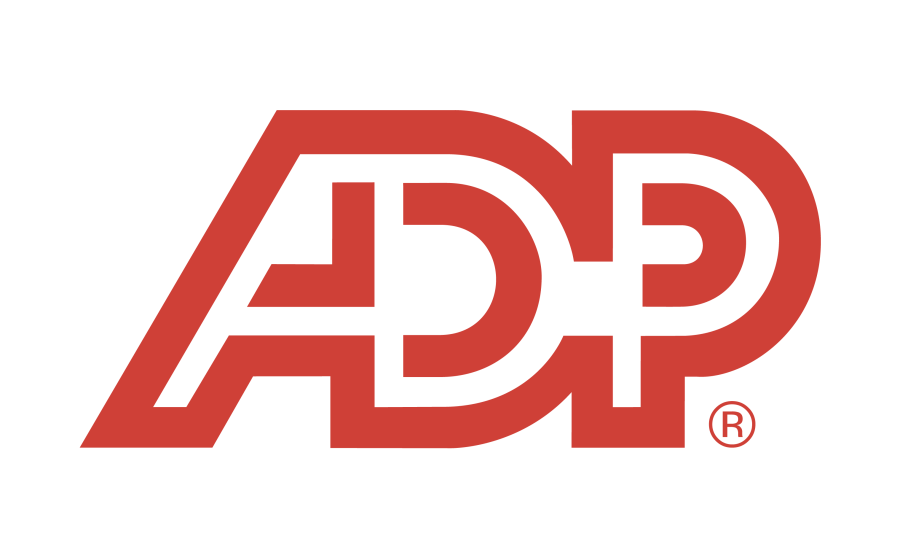


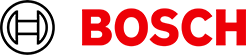
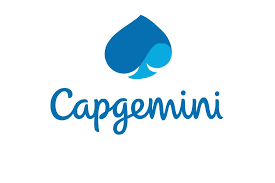
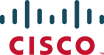


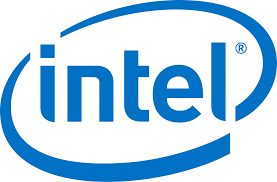

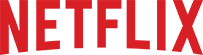
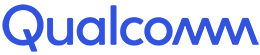
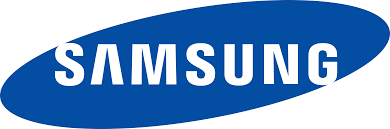


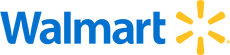
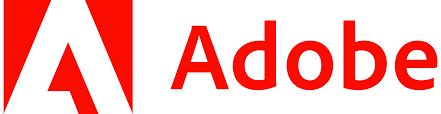
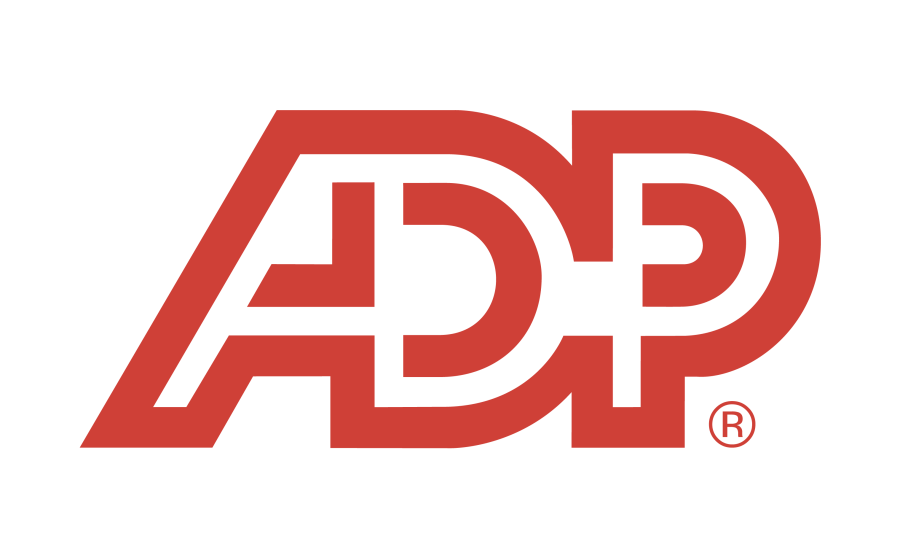


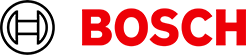
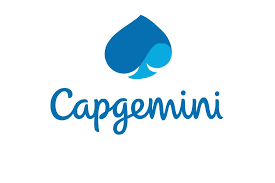
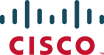


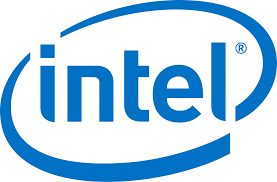

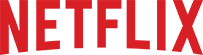
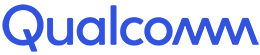
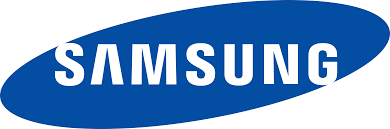


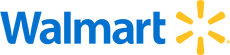
Related eBooks
Annual Membership
Become a valued member of Tutorials Point and enjoy unlimited access to our vast library of top-rated Video Courses
Subscribe now
Online Certifications
Master prominent technologies at full length and become a valued certified professional.
Explore Now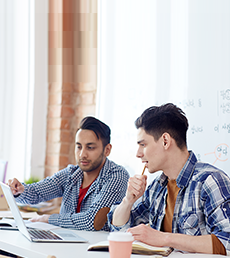